AI – with great potential comes great responsibility
As researchers continue to find applicability for artificial intelligence in healthcare, many say they want to know more before ceding away too much control to the technology.
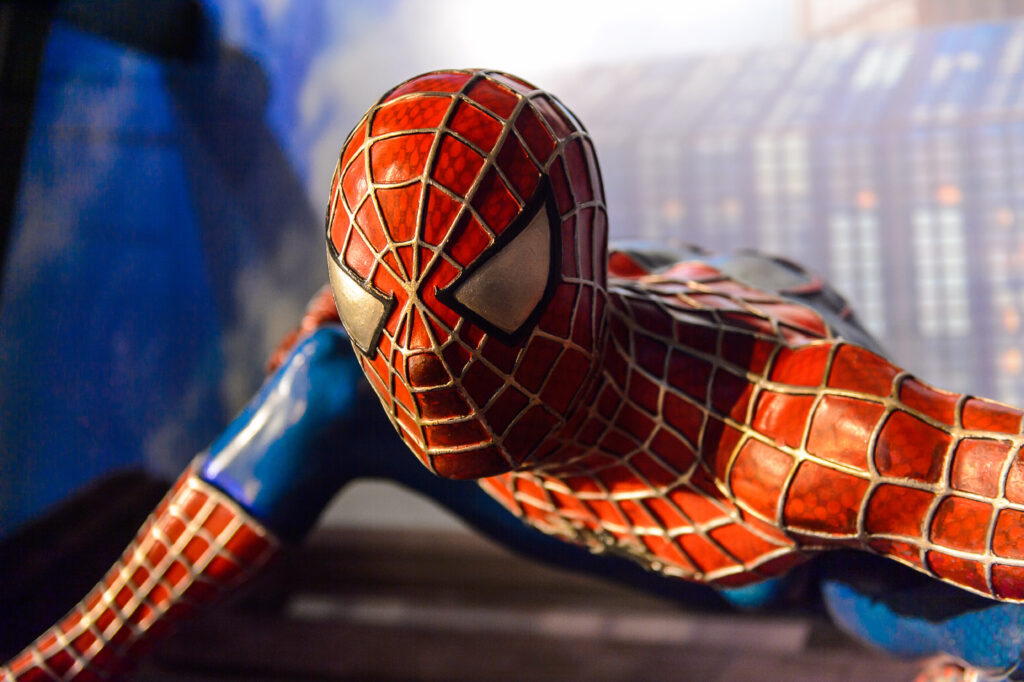
While there’s a lot of momentum behind the use of artificial intelligence in healthcare, there’s also growing concern about the need to pump the brakes on hype and expectations.
There are increasing calls for ensuring that AI is trustworthy and that the process for developing algorithms and using it is transparent.
In fact, healthcare organizations ranging from the Office of the National Coordinator for Health Information Technology (ONC) to the Future of Life Institute have called for the industry to tap the brakes a bit on artificial intelligence models to gain a better understanding of how the technology is working and building trust in the way it works.
It is indeed an unusual position for the healthcare industry to be in. Historically, the industry has been resistant, or at best reticent, to embrace change. While artificial intelligence has been available in the healthcare space for more than a decade, the hype around ChatGPT has catapulted expectations for the use of all AI in healthcare.
That, and nagging fears around the rush to use AI in healthcare has catalyzed discussion about how to safely integrate the technology into healthcare, with appropriate safeguards and trust-ensuring mechanisms.
ONC’s concerns
For its part, ONC sees AI oversight as fitting into its recently proposed Algorithm Transparency, and Information Sharing (HTI-1) Proposed Rule, which generally implements provisions of the 21st Century Cures Act and updates ONC’s Health IT certification program.
In a blog, ONC states it understands that the industry is early in the use of “predictive models, especially those driven by machine learning.” And ONC gets involved because such models often are driven by the data in electronic health records systems, leading to informed clinical decision support systems, and it proposes that predictive capabilities be covered “as part of a revised version of our CDS certification criterion.” And it seeks to ensure that users know when data relevant to health equity are used in predictive models.
Other blogs by ONC review potential risks of predictive capabilities, including amplifying structural biases of society and healthcare, reaffirming “existing, inexplicable differences in health and health outcomes,” and making ineffective or unsafe recommendations to users.
Finally, ONC proposes increasing transparent risk management for and better management for predictive decision support, seeking more public disclosure of risk management practices that address concerns related to validity, reliability, privacy and security.
Looking for guardrails
ONC’s concerns are aligning with others in the industry who see increased need for transparency to better ensure rigor to buttress AI initiatives in healthcare.
In April, the Coalition for Health AI (CHAI) published its initial version of a blueprint for trustworthy AI in healthcare. The document is intended to address the quickly evolving landscape of health AI tools, offering specific recommendations to increase trustworthiness within the healthcare community, ensure high-quality care and meet healthcare needs.
CHAI contends that its document reflects “a unified effort among subject matter experts from leading academic medical centers and the healthcare, technology and other industry sectors, who collaborated under the observation of several federal agencies over the past year.”
The coalition’s efforts are in part responding to federal calls for guidance on how the new technology will play with traditional HIT and help with concerns, such as identifying bias in output, says longtime healthcare IT leader John Halamka, MD, president of the Mayo Clinic Platform and a steering committee member for CHAI.
The concerns of providers as well as patients, payers, pharmaceutical companies and policymakers must be addressed before AI can make positive contributions in healthcare, Halamka has contended.
CHAI’s efforts, although engendering participation from federal agencies, are primarily a first stab at building consensual guidance, Halamka says. “We are not making policy; we are not a lobbyist. We aim to convene government and academia in neutral forum, then derive what best practices are and synthesize that into a set of guidance,” he explains.
The popularized use of AI in the form of ChatGPT has spurred other calls for action. Most noticeably, the Future of Life Institute in late March circulated a letter calling for a pause on the training of artificial intelligence models larger than GPT-4 for six months.
The group’s concern is that AI developers are racing to roll out technology that cannot be understood, predicted or reliably controlled. While not singling out healthcare per se, the institute’s letter contends that “AI systems with human-competitive intelligence can pose profound risks to society and humanity,” which it says has been shown by research and “acknowledged by top AI labs.” Because such developers are racing to develop and deploy more powerful AI capabilities, it’s time to rein in development until mechanisms are created to assess risks and potential protections.
“Powerful AI systems should be developed only once we are confident that their effects will be positive and their risks will be manageable,” the letter states. “OpenAI's recent statement regarding artificial general intelligence, states that ‘At some point, it may be important to get independent review before starting to train future systems, and for the most advanced efforts to agree to limit the rate of growth of compute used for creating new models.’ We agree. That point is now.”
Calls for caution in healthcare
Those calls for reassessment of AI development are warranted, and the healthcare industry should take notice, says Kay Firth-Butterfield, executive director of the ED Centre for Trustworthy Technology, who has signed the Future of Life Institute’s letter.
Taking part in a panel at HIMSS23 entitled, “Responsible AI: Prioritizing Patient Safety, Privacy and Ethical Considerations,” Firth-Butterfield notes questions remain around bias in the data fed to AI systems, informed consent, fairness, accountability and who’s at fault when something goes wrong.
“Although we talk a lot about concerns that there isn’t any regulation around AI, there is actually law out there” to govern it, she contends. “We have to think about accountability issues. If we’re going to use generative AI systems, what data are you going to share with those systems? The quality of data and where it comes from are pernicious challenges.”
Peter Lee, corporate vice president of research and incubation at Microsoft, acknowledges both the potential and concerns around AI. “There are some scary risks – there is a lot to learn. There are tremendous opportunities here, but significant risk that we don’t know about. The healthcare community needs to assertively own how to use this.”
“There are a lot of black box models in AI,” concurs Reid Blackman, the author of the newly released book “Ethical Machines” and founder and CEO of Virtue, a digital ethical risk consultancy. “ChatGPT-4 can be opaque in how it comes to a conclusion. So, what is the benchmark for safe deployment? Maybe we’re OK with magic that works – but if AI is making a cancer diagnosis, I need to know exactly why.”