How AI and ML can exponentially aid drug development
Vast stores of health data can advance findings in drug toxicity and speed drug development, transforming the therapeutic approval process.
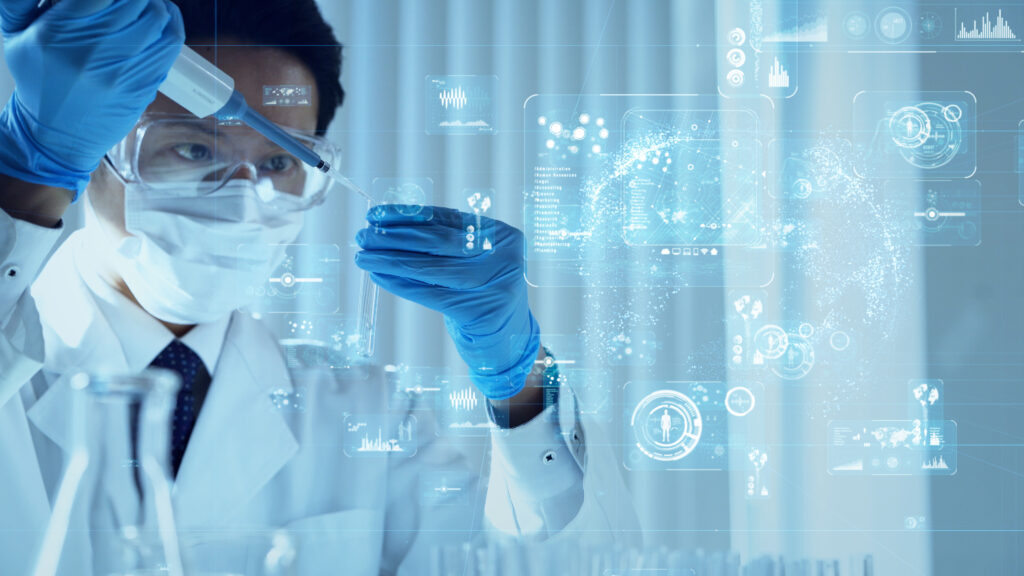
It takes 10 to 12 years for a novel therapeutic to make it through the traditional drug development and approval pipeline in the United States, and that’s if nothing goes wrong. In that time, more than six million Americans will die from incurable cancers alone.
While the Food and Drug Administration (FDA) has created multiple programs — accelerated approval pathways, compassionate use and expanded access programs, for example — to alleviate some of the associated negative outcomes of this timeline, many of the patients who need new treatments the most are left waiting.
Applied strategically to drug discovery and development, artificial intelligence (AI) and machine learning (ML) have the power to drastically reduce the time to approval and remove many of the barriers that keep treatments with potential to do good from making it to market.
Earlier this year, the FDA paved the way for the intentional and regular application of AI and ML to drug development with the publication of the discussion paper, “Using Artificial Intelligence and Machine Learning in the Development of Drug and Biological Products.” The agency subsequently requested feedback from the biotech industry. And if the growing body of evidence for AI’s and ML’s positive impacts on drug development are any indication, it’s here to stay.
AI can accelerate data analysis
Part of what makes drug discovery and development a relatively slow process is the amount of time it takes to collect and analyze data. Never has this been truer than now, when daily data creation is being measured in petabytes. International Data Corporation forecasts that the global datasphere — or total amount of data generated the world over — will reach 175 zettabytes by 2025. And data production in healthcare is projected to grow the fastest of all industries.
While that may sound overwhelming, it’s a good thing for drug development. In 2022, Dagenais et al determined that real-world evidence and real-world data could improve regulation, particularly in respect to approval for therapeutics for oncology and rare diseases. In addition, that evidence and data could also be used to help inform drug development strategy and the design of clinical trials.
When it comes to therapeutics themselves, the more data that’s available, the more we can learn about compounds and how they affect people. While analyzing viral genomes during a study on SARS-CoV-2, Fumagalli et al (2023) found that the wide availability of omics datasets from both healthy individuals and those with the disease could greatly inform the selection of therapeutic compounds.
Essentially, a larger amount of data could lead to a better and more effective treatment. However, because there is no standard template for the collection and analysis of datasets, there must be a way to wade through the large, complex and markedly different data files.
Enter AI – it’s faster than a human researcher and can support accelerated analysis of omics data from multiple sources. In 2023, researchers confirmed that AI and ML can enable researchers to collect and integrate omics data from multiple sources, and the technology can stratify that data to help pinpoint the most helpful therapeutics for varying levels of disease progression and treatment resistance.
As researchers around the world get better at using AI and ML to support this type of precision, personalized medicine becomes more possible and effective.
Advancing the global knowledge database
Sun et al (2022) analyzed clinical trial data from 2010 to 2017 to better understand why therapeutics with promise fail. The researchers found that a lack of clinical efficacy causes 40 percent to 50 percent of drug failures — the drugs didn’t do what they were supposed to do — and 30 percent were the result of unmanageable toxicity, meaning that the drugs caused adverse events or harm to patients, well above and beyond acceptable levels of risk.
With zettabytes of real-world data available, it is entirely possible to use AI and ML to build effective models that accurately predict drug efficacy and toxicity, and in stratified patient cohorts, during the drug discovery screening stage. In other words, researchers could determine how effective a specific compound will be in a particular group of patients based on their data similarities, and whether they are likely to experience adverse events, before ever giving the compound to a person.
Data from pharmacokinetic and pharmacodynamic studies are particularly helpful in accurately modeling drug reactions. While studying metabolism with multi-organ chips, Shroff et al (2022) noted that unexpected toxicity usually results from a lack of understanding of drug metabolites and the limitations of animal studies conducted prior to human studies. This is something that the FDA’s new report indicates could be resolved by the application of AI or ML.
Ultimately, reducing the risk of research and development investment pursuant to successful clinical trials is one of the most important things that AI and ML can do for drug development. Reducing the risk of clinical trials could help 30 percent more novel therapeutics achieve FDA approval and make it to market. For the millions of Americans waiting for lifesaving and life-improving drugs right now, AI and ML could be the difference between a life well lived and a life lost.
Jo Varshney is the founder and CEO of VeriSIM Life.