How AI can play a role in shaping value-based care’s future
Organizations must expand their vision for how AI can transform diagnostics, treatment pathways and patient care, marking a new era in health data management.
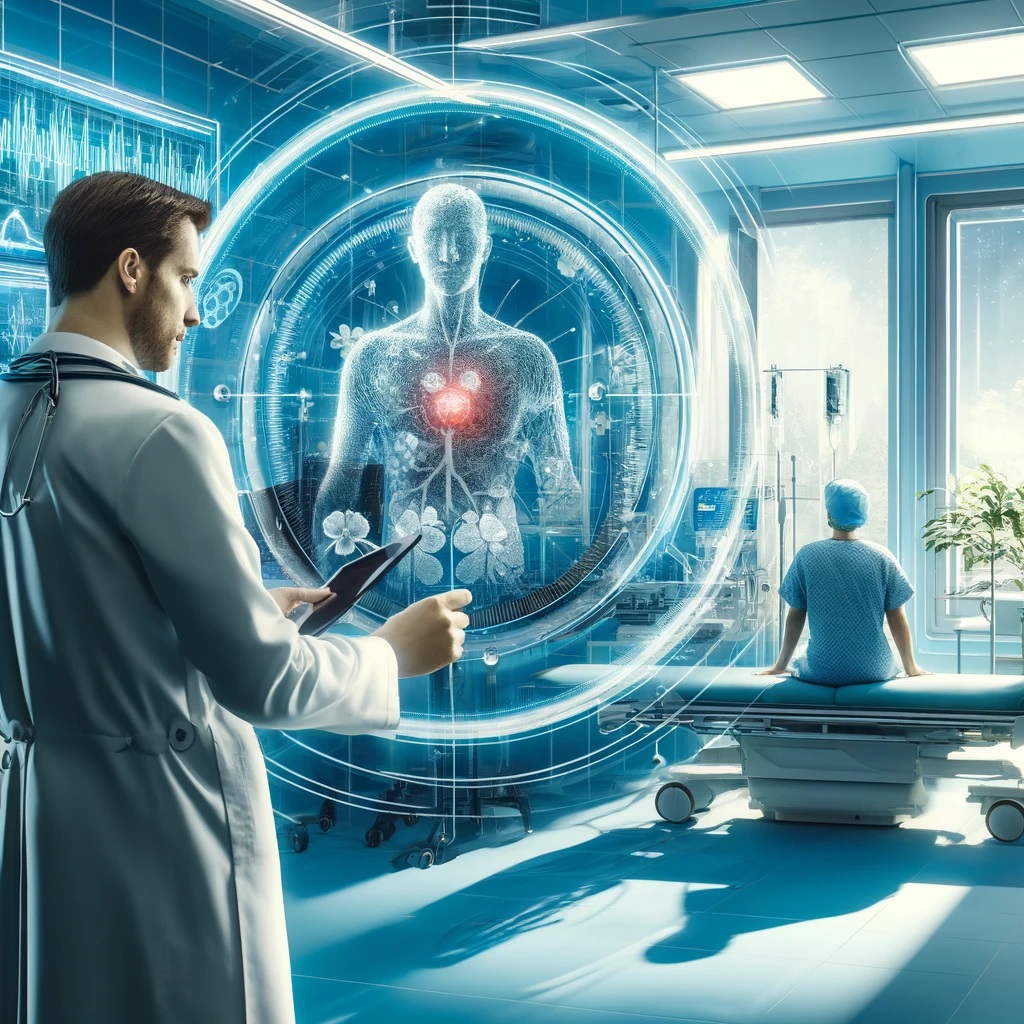
Artificial intelligence is the promised solution for just about everything wrong or missing in healthcare. From faster and more accurate diagnoses to clinical development with effective drugs tailored to patient characteristics, AI has something for everybody.
The growing availability of clinically rich patient data along with other data types will transform the potential for healthcare and of human life.
The astounding growth of AI across all specialties of medicine is daunting. Imaging has literally changed the understanding of many conditions and now enables clinicians to more rapidly diagnose and stem progression of disease. AI is popping up everywhere, from evaluating patient propensities for genetic mutations to life span prognosis. There is no area of specialty research that isn’t investigating the potential for AI to improve medicine.
By December 2023, the FDA had cleared 700 AI algorithms for healthcare, with the majority in radiology but cardiology increasing swiftly. This is no longer the stuff of science fiction, but technologies that are implemented in medical centers.
Take HeartFlow, for example, approved by the FDA in late 2022. HeartFlow is using an AI algorithm in conjunction with a cardiac CT scan to diagnose coronary artery disease to define fractional flow reserve (FFR) hemodynamic flow, replacing non-invasive technology with a procedure that was typically invasive and performed through cardiac catheterization.
Other cardiological risk assessments and diagnostic tools have been developed and are in clinical trials or used as screening tools now. Cedars-Sinai medical scientists have created an AI algorithm-assisted echocardiograph that identifies two heart conditions that are very difficult to find, hypertrophic cardiomyopathy and cardiac amyloidosis. The Echo-Net device is now in recruitment for clinical trials for cardiac amyloidosis. Mayo Clinic researchers in conjunction with a cardiac AI company, Anumana have developed an AI-assisted ECG called ECG-AI LEF to predict earlier heart failure, enabling interventions for the patient. It is now approved by the FDA.
Across many other specialties, similar progress with risk algorithms is occurring. The early identification of chronic kidney disease (CKD) has been a particularly gnarly issue for clinicians and impacts patient prognosis and costs. More than one third of people in the United States older than age 65 have CKD, and fewer than 15 percent of the total population.
But multiple risk factors and the lack of early screening tools make it difficult to diagnose early and prior to symptoms. AI algorithms have been developed that weigh various risk factors, while illuminating problems that are stalling a final accepted methodology, such as absence of data or accepted CKG risk standards in EHRs. Nevertheless, research is moving quickly to evaluate existing AI algorithms as a path to screening for early CKD.
VBC on the outskirts of healthcare
Unfortunately, the AI ideas for value-based care (for purposes here, we are focused on accountable care organizations) are different. ACOs are implementing patient-checking bots, robotic pets and assistants, Chat GPT patient communications.
There is a nod to the future of AI in predicting patient utilization. None seem transformational in the same way that medical breakthroughs enabled by AI are and are tools for use in an already-predetermined menu for value-based care. That menu in value-based care is focused on limited gains through coordinating care, keeping utilization down, and meeting regulatory requirements. Our thinking is too meager.
Transformational AI – AI-enabled medical knowledge – advances clinical development. Most important, the AI algorithms identify markers and risk characteristics in people who acquire or progress in disease. The algorithms also stratify risk of individuals in a group.
Such algorithms are not cost-focused per se because the goal is clinical, but once the code of risk factors is cracked, the probability is that future patients can reap the savings of fewer repetitive tests, procedures, provider-churning for diagnoses and disease complications. Isn’t that improved value? Why aren’t we reaping the benefits of that capability in our value-based care initiatives?
Perhaps ACOs and other entities in value-based care just don’t perceive these undertakings is part of their value-based care responsibilities. But we will never realize the value of clinical progress in healthcare outcomes, lower costs or health equity if the value and delivery structures don’t operate in tandem. Likewise, the specialty areas that have created successful algorithms for predicting risk will operate without the resources of population health or patient navigation that ACOs have.
Divided responsibilities
All ACOs are responsible for improving outcomes and keeping costs within a benchmark, while ensuring equitable delivery of healthcare services and a good patient experience. Many ACOs will acknowledge that their authority to undertake these higher goals is limited.
The lack of data in general for ACOs for completing these tasks is also well known. All ACOs receive claims data from Medicare. Even so, they are responsible for conducting improvement programs for both quality and cost, which must involve clinical data to be effective. Most ACOs don’t aggregate EHR data to identify patient risk. Many ACOs also use claims data only in “cost management,” which by content excludes all clinical information except diagnoses.
In fact, ACOs may not understand that the quality of EHR data is relevant to them, especially as few have or use it currently. ACOs as a group fought hard against the need to collect EHR data for quality reporting by continuing to report quality to Medicare on a small sample of patients. And losing that battle, most ACOs are trying to restrict data collection for that effort, either by restricting the method of reporting to Medicare patients only or by selecting a format that contains minimal data.
ACOs that will be the leaders of the future in value-based care will need to take on more. Those who participate in risk-based payment models need even more to be using predictive tools to avoid preventable admissions and other costs.
The most important functions an ACO can perform are filling the gaps between fragmented specialty care and social services and providing a holistic view and plan for the patient. These include creating the patient-centric value-based care database, sharing performance analytics, centrally coordinating improvement initiatives, and establishing referral networks to external specialists and social services.
Before they can take on those tasks, they need some strategies to go about how to build the substrate for future endeavors. Here’s a simplistic approach.
Three breakthrough strategies
Aggregate data from all providers’ EHRs in the ACO and help practices optimize their data value. Most EHRs have data reporting capability, and the ACO data aggregation vendor should be able to accept multiple file formats, including separate files of demographic, transaction and clinical data. However, data can be stored in separate tables in EHRs with customized templates, or data “stored” in progress notes. The data vendor should help ACO participants take advantage of their important data by identifying gaps and missing expected data (find the template-hidden data).
Provider tutorials will no doubt be involved. So too will visually sharing the data to convey what poor data does to analytics. ACOs must be prepared to help groups the real value of their clinical data to their patients’ care. Data is the fuel for ACO performance efforts, and data content must be continually improved.
Build the data substrate for value-based care to be clinically rich data that includes scoring from specialty risk algorithms. ACOs may be short on data, but many participating providers are not. Providers are piloting functionalities like natural language processing (NLP) into their EHRs to reduce documentation time and costs and capturing more clinical and social data. This data should be part of the aggregation.
Top medical facilities with research capabilities are using FDA-approved AI devices and software algorithms to identify and score patient risk and refer patients to services. Hundreds have been approved in radiology alone, and cardiology is ramping up. Patient risk scores should be integrated into the ACO database from separate systems, as necessary.
Share data with providers, including performance data, patients in episodes of care and general patient risk data. Providers should be able to access the shared VBC clinical records for their own patients. Providers should see where their own patients are in episode analytics, in cost variation for every episode, and compared with their other patients.
The achingly slow transition to value-based payment models has limited the transformation to value-based care in many ways, so providers have neither the data nor the experience in using data for cost or quality solutions. ACOs, which have been the champion of the Medicare value-based care program, have often not had the resources to invest in technology. But that is changing.
With the advance of artificial intelligence and of EHR capabilities, healthcare organizations have new opportunities to benefit by sharing data for the improvement of patient health outcomes. ACOs are developing innovations and learning data skills that will position them for future collaborations. It is a perfect time to conceptualize how AI can be used to bridge the divide between healthcare and its arm for demonstrating value.
Theresa Hush is CEO and founder of Roji Health Intelligence, guiding healthcare systems, providers and patients on the path to better health through solutions that help providers improve their value and succeed in risk.