How multimodal data from federated networks enables healthcare innovation
These wide-scale data networks are bridging the gap between scattered health data sources and providing insights for research and scientific discovery.
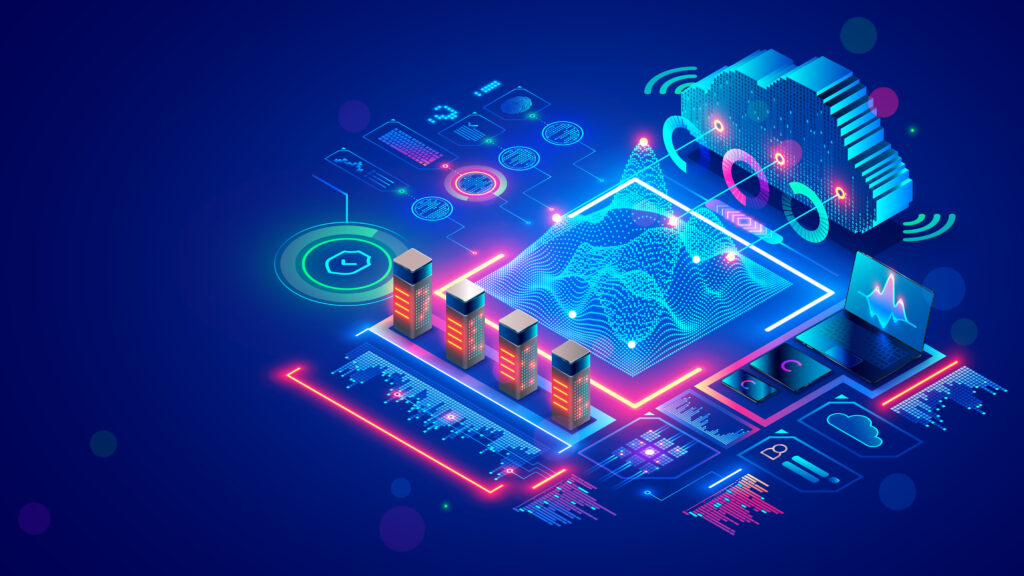
When it comes to using real-world evidence for scientific insight generation, there are a litany of challenges.
First, there is not enough data – much less diversity in the data – generated from any one health system. Insight generation requires data at scale.
Second, the data is usually limited to structured data like claims or diagnostic codes. It is seldom broad and multimodal.
Third, the rich insight-generating aspect of the data is often trapped in unstructured forms like clinical notes, raw ECG waveforms or CT scans. Artificial intelligence tools like natural language processing (NLP) and machine learning are required to extract that data.
In addition, the data typically is not located in one easy-to-access place. It usually lives in silos at academic medical centers or is sold as a specific type of modality from a third-party vendor.
Also, there are important privacy concerns when it comes to giving access to patient data for research and scientific discovery. Harnessing large amounts of multimodal data must be done in a secure and privacy-preserving manner.
Solving the scale problem
The solution for researchers – whether they work at academic medical centers or life sciences companies – is to make it easier to access and interpret multimodal data at scale. This means leveraging a federated data network that combines a scalable research infrastructure with privacy protection.
Here are six benefits of a federated data network and features to consider.
Scale. A federated data network provides the breadth of data needed for impactful research, because it comes from multiple academic centers. Researchers no longer are limited to data from within their own system, what a colleague at another organization shares or a narrow slice of data purchased through a vendor. They can access all of the data available from the partners in the federated data network. This means more diverse data from millions of health records across the country and, increasingly, the world.
Multimodal data. An important aspect of a federated data network’s value is based on the types of data available. Some networks may focus on a specific therapeutic area like oncology, or a specific type of data such as structured claims and diagnostic codes. To unlock the most value possible for discovery and development, it is critical to have as many types of structured and unstructured data as possible, including free text from the EHR, radiology imaging, digital pathology imaging and multi-omic data.
Common data schema. Without a federated data network, the data in each healthcare system is siloed and can lack interoperability for researchers. By joining a federated data network, the data is harmonized to a common data schema and accessible on a single platform and research infrastructure regardless of where the data originates.
Privacy and security. Healthcare organizations are understandably sensitive about sharing healthcare data, especially if you are asking them to share such a broad scope of multi-modal data. Utilizing best-in-class de-identification technology to protect patient privacy is critical — the goal is to obfuscate any information that could possibly identify a patient while preserving data richness for researchers. A federated data network also ensures that data is firewalled or remains “behind glass,” meaning that it never leaves the healthcare center from which it originates. It stays within the institutional firewalls while a federated infrastructure unlocks it, making it accessible for researchers via a cloud-based platform.
Ease of use. There is a real need for software tools that make it easy for researchers to derive insights from these large, complex datasets. This means using natural language processing to extract entities from unstructured data like clinical notes. Also, for researchers training an AI algorithm, they need a workflow to rapidly tag and annotate training and validation datasets at scale. By focusing on these key end-user needs, nference has developed both no-code apps to find real-world insights as well as workflows to build and validate AI algorithms.
Revenue diversification. Many hospitals are struggling with margins and looking for ways to diversify revenue. One possibility is to develop new algorithms using the federated data network’s tools, which they can then commercialize.
Federated data networks are shaping the future of healthcare innovation. By harnessing unstructured, multimodal data at scale, collaborative efforts enable more rapid research and progressive strides in precision medicine. Increasingly, strategic partnerships are being seen as a pivotal mechanism for connecting hospital systems, technology companies and life science organizations to leverage the immense potential of multimodal clinical data for research and discovery.
Alastair Blake, MD, is vice president of clinical and commercial partnerships of nference.