How to build safe, secure and trustworthy AI capabilities
While there are high hopes for this advanced technology, development depends on using caution and implementing a multi-faceted approach.
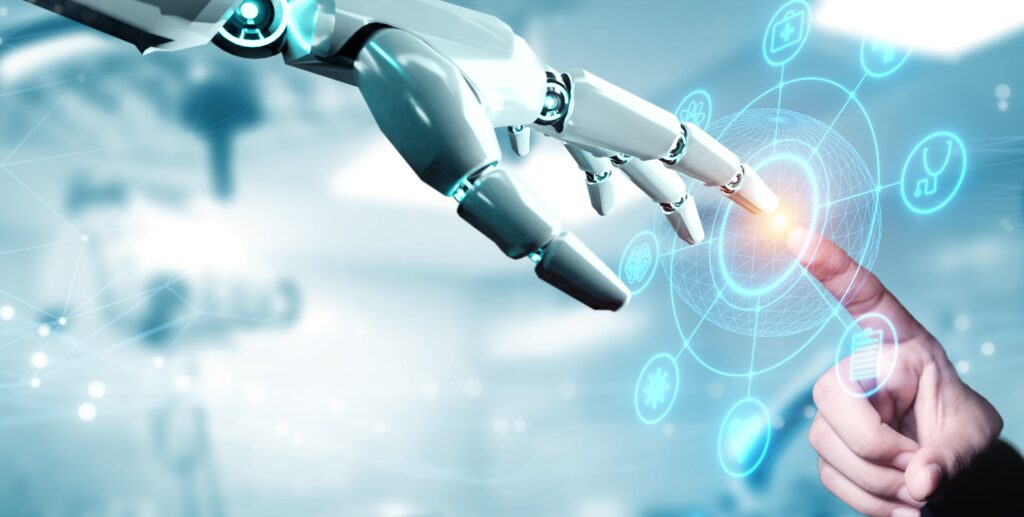
Advances in artificial intelligence are transforming people's lives in ways previously unimaginable. As AI capabilities expand, so do the implications for the safety and security of data and people.
Developing safe, secure and trustworthy artificial intelligence involves a multi-faceted approach that emphasizes ethical principles that parallel technical excellence. This involves building systems that are resilient, transparent, fair and accountable, with measures in place to reduce potential risks.
There are some key essential principles and actions for creating AI that prioritizes safety, security and trustworthiness.
Regulation and standards. It's crucial to create clear industry guidelines and best practices to ensure safety, security and fairness. This can be achieved through collaboration among governments, academia and industry. Additionally, fostering global coordination in AI governance is essential to ensure that AI technologies are developed responsibly and that important global concerns, such as data protection and human rights, are upheld.
Various government agencies are expected to lend a hand here. For example, the National Institute of Standards and Technology will establish strict red-team testing standards to ensure the safety of AI safety before it’s released to the public. The Department of Homeland Security will apply these standards to critical infrastructure and create the AI Safety and Security Board. In addition, the departments of Energy and Homeland Security will also tackle AI threats to critical infrastructure, as well as risks related to chemical, biological, radiological, nuclear and cybersecurity issues. These actions represent the most significant government efforts to advance AI safety.
Transparency and explainability. To fully trust results derived from AI, it’s important to design systems that offer clear explanations for their decisions, helping users understand AI behavior, build trust and assess the reasonableness of outcomes. It’s crucial to use interpretable models or provide tools that visualize and clarify how AI reaches its conclusions, especially in critical fields such as healthcare, finance and criminal justice.
Additionally, AI systems should be auditable, with comprehensive documentation detailing the model's architecture, training data and decision-making processes to ensure accountability and facilitate independent reviews.
Security measures for AI systems. These focus on ensuring robustness against attacks, such as adversarial manipulations that could lead to incorrect outcomes. AI systems must be designed to function properly under various conditions, including during attempts to deceive or mislead the system.
Additionally, strong encryption and secure data storage practices are essential to safeguard user data, with sensitive information like personally identifiable data being anonymized or encrypted.
Regular testing and verification are crucial to identify and address vulnerabilities, including simulating real-world adversarial scenarios, conducting penetration tests, and applying formal verification techniques when needed.
Fairness and bias mitigation. Concerning AI, this involves conducting regular audits to identify and address biases in datasets and models, ensuring that AI systems do not reinforce or amplify existing societal biases related to race, gender or socioeconomic status.
It is essential to use diverse and representative datasets when training AI models to ensure fair representation of various demographic groups. Additionally, fairness constraints should be integrated into the development process to prevent discriminatory outcomes, particularly in sensitive applications such as hiring, loan approvals or law enforcement decisions.
This article explores the use of AI in research, emphasizing key ethical concerns and offering several recommendations for responsible use.
Accountability and governance. As this intersects AI, it requires establishing clear responsibility for developers, organizations and stakeholders regarding the behavior of AI systems, especially when these systems make significant decisions. It is crucial to ensure that AI systems comply with relevant laws and regulations, such as data privacy laws and consumer protection standards. Additionally, implementing continuous monitoring mechanisms is essential to track the performance of AI systems, ensuring they function as intended and enabling adjustments when unforeseen issues emerge.
In their paper, Cussins, Newman and Oak identify key challenges related to AI, grouped into four areas – design, process, use and impact. The design area covers decisions on what to build, how and for whom. The process area addresses supporting transparency and accountability through institutional design. The use area highlights how AI can be misused to harm individuals or groups. Lastly, the impact area explores the broader social, political, psychological and environmental effects of AI technologies.
Ethical and responsible AI design. This requires aligning AI systems with human values and societal goals, incorporating principles such as fairness, privacy and non-discrimination. It is essential to ensure that AI enhances human decision-making rather than replacing it, with human oversight and control in key decision points. AI should function as a decision-support tool, ensuring that humans retain final control over critical decisions, especially in situations where safety is paramount. Promoting interdisciplinary collaboration among ethicists, social scientists and policymakers is also vital to ensuring the ethical integrity of AI systems.
This paper highlights three key points — autonomy, the right to explanation and value alignment — to emphasize that ethics should be embedded within the systems themselves, not merely serving as principles for guiding users. While AI presents broader challenges, the author argues that machines, as tools in the first machine age, were guided by human ethics. In the second machine age, with AI mimicking human-like processes, ethics must be embedded within the systems, positioning machines not as moral subjects but as moral agents. This does not equate machines to humans, but calls for a new ethical framework in the era of AI and human coexistence.
Anthropomorphizing AI with traits like "ethics" creates unrealistic expectations of inherent ethical behavior. Instead, the authors call for more research on the ethical use of AI throughout its lifecycle, from design to operational use. They propose key research areas — ethics and AI education for developers, leaders and users; using model cards or datasheets to provide transparency on datasets and model biases; human-centered design for effective human-AI interaction; run-time assurance to enforce ethical principles like safety and bias reduction; and best practices for joint human-AI co-creation to improve performance and align mental models.
Privacy protection. In AI, this involves minimizing the collection and processing of personal data, ensuring that only the essential information needed for the system to operate is gathered.
Without proper safeguards, AI can put people’s privacy further at risk. Privacy-preserving techniques, such as federated learning or differential privacy, should be used to enable AI models to learn from data without compromising sensitive information. Additionally, transparency is crucial — organizations must clearly inform users about how their data is collected, utilized and stored, and obtain explicit consent for its collection and usage.
Continuous learning and adaptability. This capability in AI requires ongoing maintenance and updates throughout the system’s lifecycle. This includes regularly re-evaluating models, retraining them with new data, and incorporating feedback to enhance performance over time.
AI systems also must also be able to adapt to evolving environments, regulatory standards and societal expectations. Achieving this adaptability necessitates agile development practices and the ability to quickly respond to emerging issues or risks.
Public engagement and collaboration in AI. Actively involving stakeholders, including the public, in conversations about the development and impact of AI is crucial for success. Open discussions on AI ethics and governance foster trust and ensure that a wide range of perspectives are taken into account. Additionally, promoting cross-disciplinary collaboration between technologists, ethicists, policymakers and other fields is crucial to ensuring that AI solutions are socially responsible and benefit society as a whole.
Promoting innovation and competition
This country is already a leader in AI innovation, with more AI startups raising first-time capital. AI research can be catalyzed across the country by piloting the National AI Research Resource, which will provide AI researchers and students with access to essential resources and data, while also expanding grants for AI research in critical areas like healthcare and climate change.
A fair and competitive AI ecosystem can be promoted by offering access to technical assistance and resources to small developers and entrepreneurs, and otherwise supporting small businesses in commercializing AI breakthroughs.
As AI continues to evolve, scientists, academic institutions, funding agencies and publishers must regularly update their knowledge, ethical guidelines and policies to reflect AI's growing impact on research. These guidelines should be revised periodically as AI becomes increasingly integrated into scientific practices and researchers adapt to its use.
Given the societal impact of science, public engagement in these discussions is essential for the responsible use and development of AI in research. By incorporating these principles, AI systems can be developed and implemented to maximize their advantages while minimizing potential risks. Striking a balance between innovation, safety and trust demands continuous focus and flexible governance.
Bhavini Kaneria is a senior analytics manager and leader in informatics, machine learning and artificial intelligence. She has worked extensively on various research-based healthcare projects in Medicare, Medicaid dual eligible special needs plans and community care. She also worked on the IHARP project as a research assistant performing prediction, forecasting and data analysis. For more information, contact bhavini.kaneria@gmail.com.