How to minimize the impact of a data ‘boom’
Key strategies can help ensure data integrity, anticipating challenges both before and after a data incident.
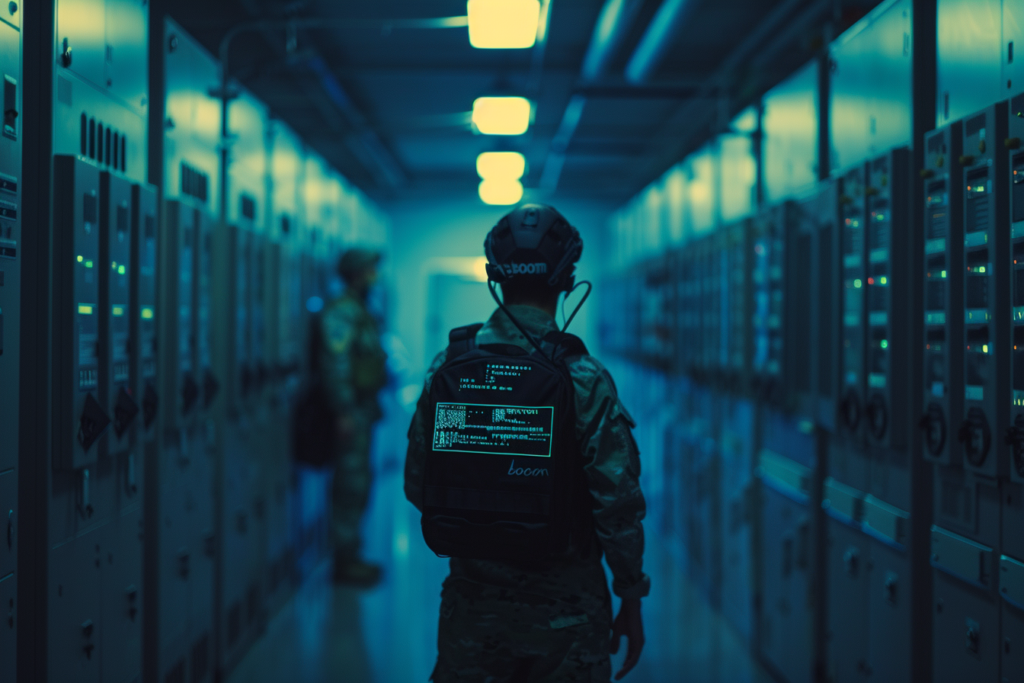
Imperfect data enters healthcare databases every day, many times a day. In fact, improving data quality was top of mind for 76 percent of healthcare leaders who responded to a recent survey.
In the same survey, 81 percent of healthcare leaders said improving data quality is a must-have for any data analytics platform they would consider. This makes sense, particularly when bad data can cause systems to come to a screeching halt.
Borrowed from the military and cybersecurity, “boom” describes a significant, disruptive event — such as bad data accidentally entering a system. The military also has a framework to categorize strategies that prepare for and react to these events — left of boom and right of boom. Left-of-boom strategies entail prevention, whereas right-of-boom strategies refer to how systems react to a major data issue. Here’s how you can apply these tactics to your data quality operations so your teams can continue delivering on the promise of healthcare, even in the event of a “boom.”
Proactive strategies: Left of boom
Healthcare leaders can use left-of-boom strategies to build a stronger, more resilient system with fewer errors. From leveraging automation to thorough documentation, here’s how to build processes that filter and deter bad data.
Prevent human error. First, automate repeated tasks. Use automation to reduce human error. For example, rather than having a human upload files to a directory on a regular basis, manage file delivery through an SFTP (secure file transfer protocol)-compliant process that’s programmed or automated (not manual).
Then, document clearly and create checklists. Where automation isn’t possible, create specific steps for each type of data activity and establish a clear format for capturing desired configurations and outputs.
Understand what to expect in the data. It’s crucial to get clear on expected input and output. Understanding expected data configurations — whether volume (expected size of population), latency (expected every other Thursday) or context (immunization records with Current Procedural Terminology (CPT) codes) — is key to building and maintaining systems that respect these patterns.
It's also key to ensure data change transparency. Healthcare organizations should communicate changes to file formatting or content in advance. Then, leadership should assess the potential impact of these changes before they’re made and take steps to prepare for them.
Design systems that fail gracefully. Here, one key is to stop bad data at the door. Establish clear criteria for rejecting bad data at a system’s doorstep to make it difficult for offending data to load.
Then, single out problematic rows with automation. Wherever possible, isolate bad rows without blocking entire files. Consider leveraging tools that can automatically attempt a formatting correction on the problematic row, then retry the ingestion of the entire file.
Reacting to an accident: Right of boom
Let’s say that, despite all the processes above, a “boom” has rocked a data system. The earlier systems can detect a problem, the faster they can address it, minimizing users’ loss of trust. Here are some steps experts can take to act quickly and effectively when data goes awry.
Detect when something has happened. This begins with the notion of observing incoming and outgoing data. With a microscope on the data moving through a system, analysts can detect anomalous patterns. To do this, use historical trends to establish a solid litmus test for what “good” data looks like, so an automated process can easily compare these data sets against each other.
Contain the issue to minimize impact. Incidents happen, so be prepared to deploy data “seatbelts.” I’ve written previously about data seatbelts, a core component of any boom response. These are mechanisms to stop the flow of bad data so downstream users can be protected from the impact of an issue.
Restore the system to a working state. When an issue occurs, backtrack on bad data. Even if you’ve been able to isolate an issue, it’s important to be able to quickly replay data in the system, enabling incremental data to continue trickling in. This might include requesting corrected files — like a monthly claims package from a payer that was initially missing diagnosis-related group (DRG) codes — then loading that regenerated file into the system.
Then, a key step involves investing in hub-and-spoke architecture. Data platform models that operate as a true hub and spoke enable rapid replays of data through the system because every architectural component is connected to the same central model.
A healthcare system runs on accurate data, and a lapse in data quality can grind insights to a standstill. But with a solid plan in place before and after a data boom, it doesn’t have to cause a crisis, despite the inherent complexity of healthcare data. Left or right of boom, a solid detailed strategy helps prevent accidents and build trust, so an organization can rely on its data to power healthier lives and efficient delivery of care.
Mary Kuchenbrod, vice president of data operations for Arcadia.