How to plan for better data quality in healthcare
Four new considerations that data professionals need to take into account as they assume responsibility for new EHR implementations.
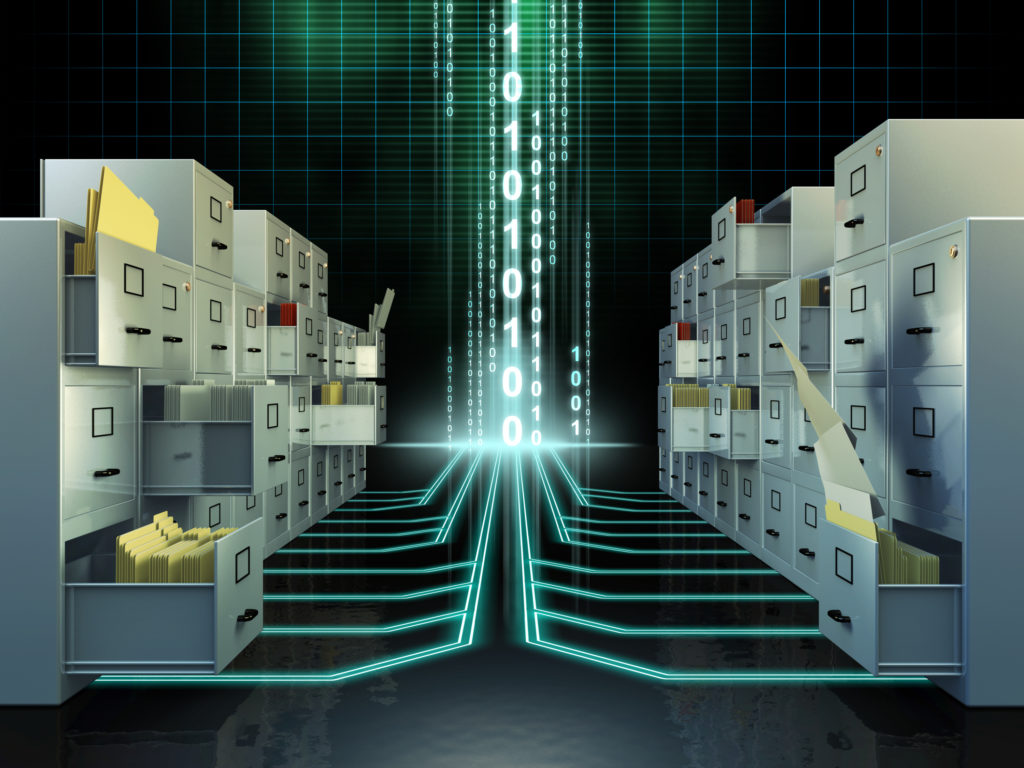
The long-term success of any new EHR system is directly correlated with the level of clinical data quality contained within.
Higher levels of data quality improve clinician satisfaction, while faulty data quality causes frustration, abrasion and efficiency challenges. Furthermore, the negative impacts of poor data quality grow as EHR data is increasingly relied upon to inform AI and digital automation.
And yet, clinical data quality is often overlooked during EHR implementations and other major system changes. In my previous article, I shared five components of a comprehensive patient data quality management plan to consider when implementing a new EHR. Here, we’ll delve deeper into the first, and perhaps most important, step: data strategy and planning.
Concerns about data
Recently, e4health completed a survey in partnership with the College of Health Information Executives (CHIME). The participating respondents were CHIME members with IT leadership responsibility within their provider organizations.
The survey revealed two specific concerns related to data strategy and planning. Some 16 percent of the responding health IT professionals implementing a new EHR relied solely on their vendor to develop a data migration strategy and plan. Also, 25 percent acknowledged not having defined guidelines for the migration and retention of legacy systems data content.
As noted in my previous article, relying on vendors to “own” the plan for optimal data quality during new system implementations is an unrealistic expectation. EHR vendors are a supporting contributor and advisor to the data quality plan, but ownership of data quality remains with the provider organization.
Here are four recommended steps to ensure a successful implementation plan focused on long-term clinical data quality.
Use Lean before implementation kickoff
Successful EHR implementations apply Lean techniques to break down large project plans into stepwise and manageable components. This step should be taken before project kickoff. The implementation clock starts ticking immediately following kickoff. Afterward, there will be added pressure to stay on schedule, which is often an excuse to de-prioritize clinical data quality.
Consider the following Lean best practices to build an effective data migration and clinical data quality management workplan before project kickoff.
Current state. Inventory existing systems, data types maintained within them and uses/user constituencies dependent on those systems and data.
Future state. Define what “done” looks like for your future state systems platform and determine where existing, historical data will reside within that future state. Define the platforms and workflows your various “user” constituents will utilize to obtain access to historical data in your future state.
Gaps and countermeasures. Map current state to future state to uncover gaps that were not previously considered.
Metrics. Define key metrics that your organization will designate to monitor and measure success. A foundational example is defining the threshold your organization will target to manage potential duplicates within your master person index (MPI).
Some vendors establish these MPI targets for you, setting a low water mark threshold for data quality. However, our experience suggests a more aggressive MPI duplicate threshold is optimal.
Another example of best practice metrics includes clinical content migration validation levels. Organizations will vary on establishing a cost-confidence curve, which is the balance between investments in the depth of their data validation efforts vs. the risks of content being migrated in error (for example, content associated to the wrong patient, incorrect mapping of historical medications or lab results).
The result of going through this process in a structured, disciplined way is the creation of an action plan framework that serves as the foundation for your data migration and management workplan. This workplan complements the overall system implementation workplan and program to support higher levels of clinical data quality.
Assess legacy systems and data
Failure to establish a roadmap for legacy system data is another planning gap often experienced during new EHR implementations.
Financial plans and expectations are often missed during new system implementations. It is during this phase that decisions should be made regarding which legacy systems will continue to be supported and maintained for historical look-up of content that has not been migrated to a future state platform, such as the EHR or archive platform.
Incorporate the following four considerations as part of your EHR implementation plan to determine the appropriate destination for legacy data and to avoid paying maintenance fees for sunset systems.
- • Map out system roles to identify remaining data and new data that will be introduced.
- • Assess archive platforms that are currently in place or may be introduced or included as part of your future state solution.
- • Identify legacy systems that will be retained and budget costs for ongoing maintenance. Also ask legacy vendors to define future dependencies for data access or extracts.
- • Evaluate the current state of your data, and the legacy systems in which they reside. Can the data be extracted in a way that retains quality and ensures discrete loading into future state platforms?
Two additional upfront gaps to consider are data governance and resource planning.
Governance and resource considerations
Data governance and resource budgeting for data quality and migration are often overlooked during EHR planning. Health IT leaders must be transparent and collaborative with teams to develop governance guidelines, share data migration plans and align stakeholders early in the implementation process.
Failure to do so early enough results in frustration, delays and budget overruns as stakeholders discover what legacy data will and will not be available in the new platform. The unexpected need to rehash these data decisions closer to go-live disrupts system plans, adds clinician friction with implementation teams, and results in unexpected data migration costs.
Many factors are involved in defining the data guidelines and principles that an organization will use as part of the data migration and long-term management plan. These include ease of legacy data access for physicians, health information management and revenue cycle.
Effective data governance planning balances the cost-confidence curve of clinical data quality by asking three questions.
- • What is the organizational tolerance for trying to achieve an ideal state of large volumes of legacy data accessible by departmental teams within the new EHR versus resource and time limitations to migrate and manage this data?
- • What data is needed on day one vs. what can be migrated to future state systems post-live stabilization?
- • What is the resource cost to validate clinical data quality in our new systems?
EHR vendors generally do a good job in outlining resources that are needed for system implementation. However, because those vendors do not own the responsibility for the quality of legacy data, the efforts around the data migration and management plan are often under-scoped and under-estimated.
Go beyond ETL, move to ETLV
Extract, Transform and Load (ETL) is the industry term commonly used to define data migration into new EHRs and other systems. However, we encourage the addition of a data validation step to ensure proper attention and resourcing is devoted to clinical data quality.
Consider extract, transform, load and validate (ETLV) as the preferred process during your EHR implementation or other major system change. The overall process of ETLV should be driven and owned by the healthcare organization, not the EHR vendor.
The EHR vendor will likely be an owner of key steps within that process (for example, load of prepared structure content into their future state platform). However, it is unlikely that the vendor will take responsibility for all four steps of the ELTV process – nor should they be relied on to own that full process.
Data validation steps include validation of technical processes and transformation mapping. Teams must also validate that data landed and is presenting as expected in the future state platforms. Proper planning for ETLV in project timelines and resource budgets is essential.
Ensuring the highest levels of data quality during EHR changes has never been more crucial. Accurate and complete patient data is the backbone of EHRs — essential for patient safety, informed clinical decision-making, end-user satisfaction, and staff productivity.
Health IT leaders are encouraged to invest time in quality data planning before the EHR vendor’s kickoff activities begin. You can rely on your EHR vendor to support data quality, but responsibility rests on your shoulders. The healthcare organization must be the owner of the data quality plan.
Jim Hennessy, FACHDM, is the president of e4health, a consulting firm specializing in healthcare IT.