Incorporating AI in healthcare: A trust-building approach
Taking steps to demystify the ‘Black Box’ can make artificial intelligence accessible and beneficial for clinicians.
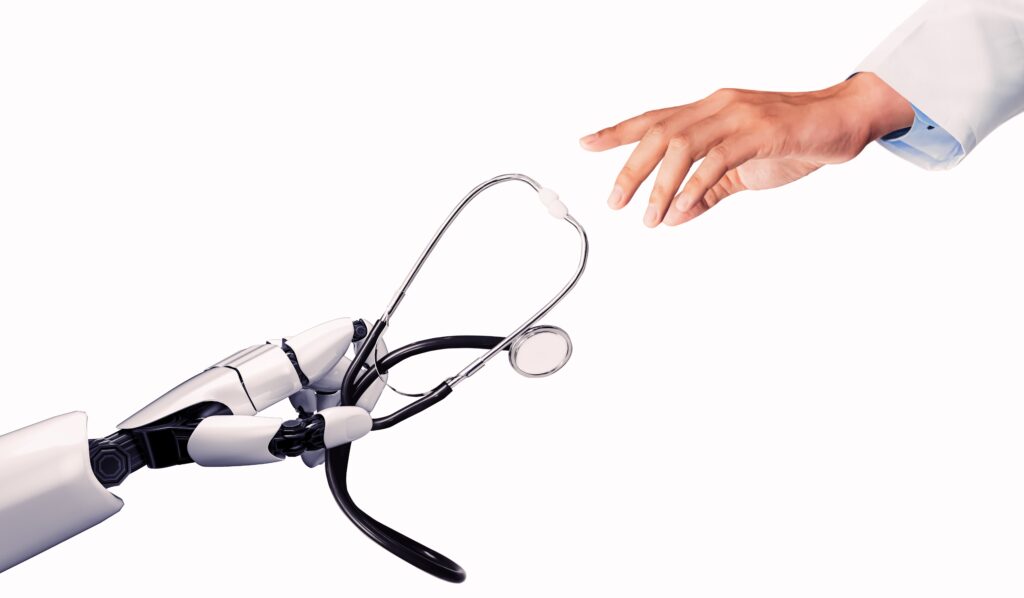
Successful patient outcomes often hinge on clinicians' ability to gather relevant data to inform clinical decisions. Although treatment guidelines may present multiple pathways for a single diagnosis, physicians often deviate from guidelines to craft personalized care plans that account for the nuances of each patient's individual circumstances.
Unfortunately, this critical data is typically housed in EHRs whose design does not support clinical workflows.
In complex disease states like oncology, it's not feasible for clinicians to review all relevant literature and analyze every data point for each patient, an exercise necessary to realize truly personalized treatment decisions. As a result of sub-optimal tooling and the demands of healthcare, modern-day clinicians are working in a mentally taxing environment in which they are expected to make life-and-death decisions without full confidence because data availability is not optimal.
The healthcare sector's embrace of artificial intelligence has the potential to reshape the industry and mitigate numerous challenges clinicians face. Notably, AI’s ability to analyze enormous amounts of data, uncover hidden insights and elevate the clinician’s ability to deliver efficient, personalized care to improve patient outcomes is undeniable.
A study by researchers from the University of Arizona Health Sciences revealed that, while patients are just about evenly split on using AI for diagnosis, clinician support of the technology can improve trust. As the number of applications in healthcare multiplies and technology matures, both healthcare professionals and patients are voicing skepticism around its safety and efficacy.
Building clinician trust
Clinicians who lack data science training may view AI as a sort of "black box," surrounded by unknowns that hinder its adoption. To alleviate concerns surrounding AI, it is crucial to provide model outputs that users can easily understand.
Interpretability is a key component of a responsible AI system, ensuring and promoting privacy, usefulness, safety, resilience and fairness while managing bias. As a result, designers, software engineers, and AI practitioners must collaborate to develop interfaces that possess a deep understanding of clinical workflows, and how clinicians access and use data to maximize useability, utility and adoption.
Critical components of these interfaces include:
- • An intuitive user experience that guides them to the intended use.
- • A clear presentation of the relevancy of training data to that patient
- • An explanation of the influential drivers and biases influencing the AI model's outputs.
By delivering AI in this way, clinicians will be more likely to understand this transformative technology, embrace it and use it to its potential – achieving the ultimate goal of improved patient outcomes.
Enhancing user experience with AI
The clinician user experience is a central yet often overlooked aspect of technology design. In complex disease states like oncology, clinicians use workarounds, such as taking notes on scrap pieces of paper, to avoid using sub-par tools.
Optimizing the experience of clinicians when designing solutions can lead to meaningful results such as reduced cognitive burden, decreased attrition and workforce turnover, improved operational efficiency and greater collaboration among healthcare providers.
Technology solutions must display the inherent basis of the outputs to instill trust in AI models among clinicians, break down the makeup of data inputs and highlight the most impactful clinical factors. Delivering these details empowers clinicians to understand the outputs and determine the correct course of action. This glimpse into the data enables clinicians to quickly understand and analyze the model to make quick, confident treatment decisions.
While the human-centric display of inputs and outputs is essential to the adoption of AI, complete interpretability hinges on the use of accessible language. Explaining model outputs in data science jargon would prevent clinician comprehension and create a sense of distrust. The nuance of speaking to the intended audience when explaining AI models is a point that cannot be ignored.
Take the following example of an explanation where an instance where the language is tailored for a data science publication is contrasted next to an explanation provided by an evidence-based clinical decision support solution.
- 1. This risk index is based on a variational autoencoder K-nearest neighbor (VAE-kNN) algorithm. The latent feature vector from the VAE was fine-tuned with contrastive learning. Absolute risk is derived from patient neighborhoods in a reference population (e.g. 9,052 patients at your facility).
- 2. This risk index has analyzed 9,052 patients at your facility. We found 200 patients with similar characteristics that we have used as a reference for this patient. This risk prediction is based on the outcome of those 201 individuals.
The comprehension and absorption of the information presented between the two statements depend solely on the audience’s data science knowledge, even though the intended message is identical. This highlights the need to intentionally develop clinically accessible language to build trust and improve interpretability.
Using interpretable AI
Any clinician-facing technology must enable, and not limit, how clinicians think and work. Current systems fall short, resulting in an overwhelming amount of data, hundreds of clicks within the software interface and time wasted hunting for information. Technology should afford physicians and nurses the mental space to do what they love – providing quality care – not forcing them to shape their workflows around an unoptimized tool.
Christine Swisher, PhD, is the chief scientific officer for Ronin.