Precision oncology and biomarkers are changing our understanding of cancer
Finding important data on patients is being aided by using artificial intelligence and machine learning to mine electronic records.
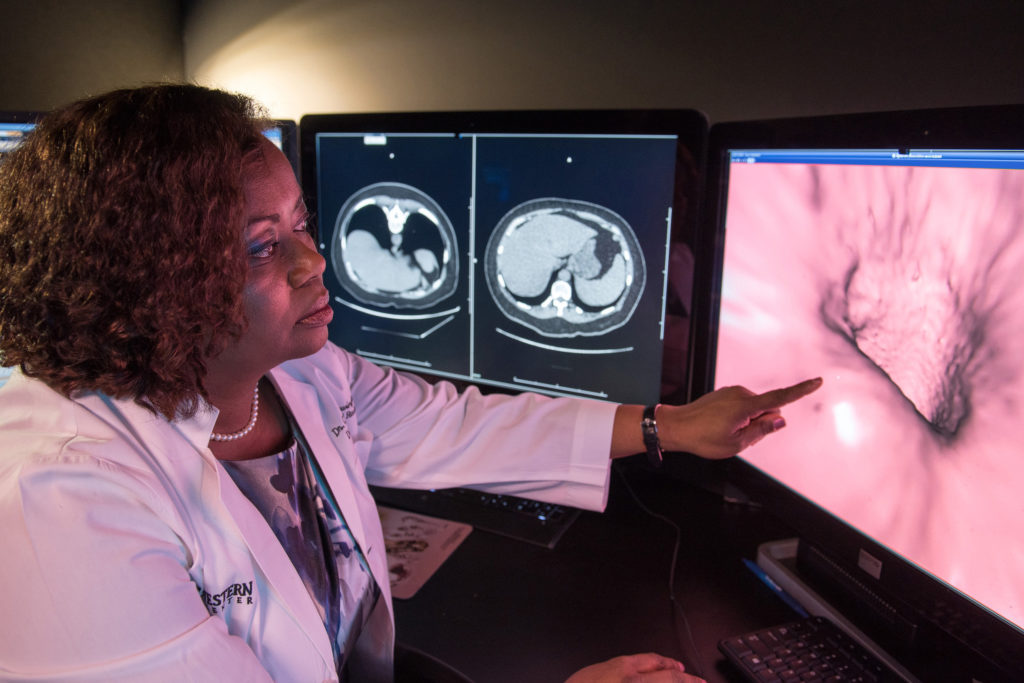
Precision oncology is transforming cancer care through personalized interventions and treatments, driven by advancements in molecular diagnostics.
Biomarker testing, a crucial component, analyzes genes and proteins in cancer cells, offering insights into an individual's specific cancer. This knowledge informs targeted strategies for diagnosis and treatment, with the potential to improve outcomes.
Biomarker testing is becoming an increasingly important step following a cancer diagnosis, as it can help clinicians better understand the specific cancer and how to treat it.
Biomarkers can be classified as prognostic, which provide insights into disease outcomes, and predictive, which evaluate potential drug responses. The use of biomarkers in cancer care is becoming more widespread, and today the National Comprehensive Cancer Network (NCCN) recommends patients with advanced or metastatic cancers have biomarker testing to help identify the most effective therapies. Biomarker testing is also indicated in some early-stage cancers. McKesson has made a strong commitment to improving patient access to testing by implementing many innovative technology solutions, resulting in a near doubling of comprehensive testing rates across The US Oncology Network.
Biomarkers and precision medicine in practice
Use of biomarkers in clinical practice can help identify which patients are likely to respond to specific therapies, thereby enhancing treatment outcomes while minimizing unnecessary side effects.
For example, the identification of genetic mutations such as EGFR in non-small cell lung cancer has led to the development of targeted therapies that have improved survival rates compared to traditional chemotherapy. Additionally, the use of biomarkers like PD-L1 expression has been instrumental in guiding immunotherapy decisions, leading to substantial improvements in response rates in certain patient populations.
As a result, precision medicine is not only reshaping treatment paradigms but also fostering a deeper understanding of cancer biology, paving the way for innovative therapeutic approaches. This evolution in cancer care is well-documented, highlighting the remarkable improvements in patient outcomes associated with biomarker-guided therapies across various cancers.
Challenges and opportunities of precision treatments
Precision treatments in oncology present both significant opportunities and formidable challenges.
One of the primary challenges is the increasing specialization of cancer therapies, which often leads to a narrowing focus on specific subtypes based on biomarker identification. As cancers are classified into increasingly specific subgroups, the patient populations for each subtype become progressively smaller. This can be particularly problematic for patients with rare cancers, where clinical data supporting treatment efficacy may be limited.
The rarity of subtypes complicates clinical trials, making it difficult to gather sufficient evidence to inform treatment guidelines. However, this challenge also presents an opportunity to leverage real-world evidence (RWE), which can provide valuable insights from individual patient experiences, helping to identify effective treatment strategies even in small populations.
The landscape of rare cancers is complex, as they collectively account for a significant portion of cancer cases — approximately one in four. Despite their low prevalence individually, the aggregation of data from these patients can yield statistically significant trends that inform treatment decisions. The use of RWE enables researchers to analyze diverse datasets and uncover actionable insights across different cancer types and treatment combinations.
By focusing on shared clinical features and biomarkers, oncologists can enhance their understanding of treatment effectiveness and side effects, ultimately leading to better-informed therapeutic choices for patients with rare cancers. This approach not only aids in the identification of effective treatments but also fosters collaboration among researchers and clinicians working with these unique patient populations.
Beyond that, the exploration of cancer mechanisms has opened new avenues for precision treatments targeting rare cancers. While distinct cancer types may exhibit unique characteristics, they often share overlapping actionable biomarkers that can be exploited for targeted therapies. This overlap enables oncologists to apply successful strategies from one subtype to another, potentially improving outcomes for patients with rare malignancies.
Additionally, as more data are collected and analyzed from broader patient groups, the potential for discovering effective treatment combinations increases. By harnessing the power of RWE and focusing on the intricacies of tumor biology, precision medicine in oncology can continue to evolve, offering hope for improved management and outcomes for patients facing rare cancers.
Strategies for adjusting research in rare cancers
To effectively study rare cancers, researchers must optimize data from small populations through several key strategies. Utilizing large data sources helps maximize patient sample sizes, ensuring statistical significance in analyses. Designing studies with appropriate endpoints and adequate follow-up time is crucial for differentiating treatment effectiveness.
Incorporating biomarkers into study designs ensures that the most suitable patients are included, enhancing the likelihood of demonstrating efficacy. Also, verifying data completeness ensures the validity of data included in analyses, maintaining the integrity of research outcomes. These approaches collectively enhance statistical power and precision, informing and establishing best practices even for the rarest cancer types.
Increasing awareness of the limitations in clinical trial representation has underscored the importance of RWE in supporting novel therapeutics. Clinical trial participants are carefully selected to minimize complications and maximize the chances of seeing a therapeutic effect, if one exists, often resulting in younger patients with fewer comorbidities and higher medication tolerances. RWE, derived from routine patient care, addresses these gaps by providing insights into treatment outcomes in broader, more diverse populations. This approach helps clinicians and policymakers assess the safety and effectiveness of treatments in real-world settings, ultimately improving the applicability of research findings to a wider range of patients.
The value and integration of real-world evidence
The increasing importance of RWE is reflected in its incorporation into regulatory decisions. From January 2019 to June 2021, 85 percent of FDA-approved applications for new drugs utilized RWE in some form, with 48 percent indicating that RWE influenced the final decision. Regarding oncology products, these rates were 70 percent and 37 percent, respectively.
This trend highlights the growing recognition of RWE as essential from both practical and regulatory perspectives. Electronic health records play a crucial role in facilitating easier access to these broad datasets.
Despite its potential, RWE faces challenges in data collection and utilization. A significant portion of healthcare data remains unused because of its unstructured format, requiring substantial processing and analysis. To address this, researchers are integrating artificial intelligence and machine learning technologies to extract meaningful information from unstructured data.
Additionally, the FDA has published draft guidance in 2025 entitled, “Considerations for the Use of Artificial Intelligence to Support Regulatory Decision Making for Drug and Biological Products”, aiming to enhance the accuracy and usability of RWE for future research efforts.
In summary, the incorporation of RWE and biomarker testing in oncology research offers a significant opportunity to improve treatment strategies for cancer patients, including those with rare subtypes. Utilizing RWE along with technologies such as artificial intelligence and machine learning can enable researchers to analyze extensive healthcare data to identify treatment trends and effectiveness across various patient populations.
Zhaohui Su, PhD, is vice president of biostatistics, Amy K. O’Sullivan, PhD, is senior vice president and chief scientific officer, and Nicholas Robert, MD, is chief medical officer at Ontada.