The role artificial intelligence and machine learning can play in cutting costs
Providers are beginning to demonstrate how artificial intelligence, machine learning and data science can reduce the cost of care while improving outcomes.
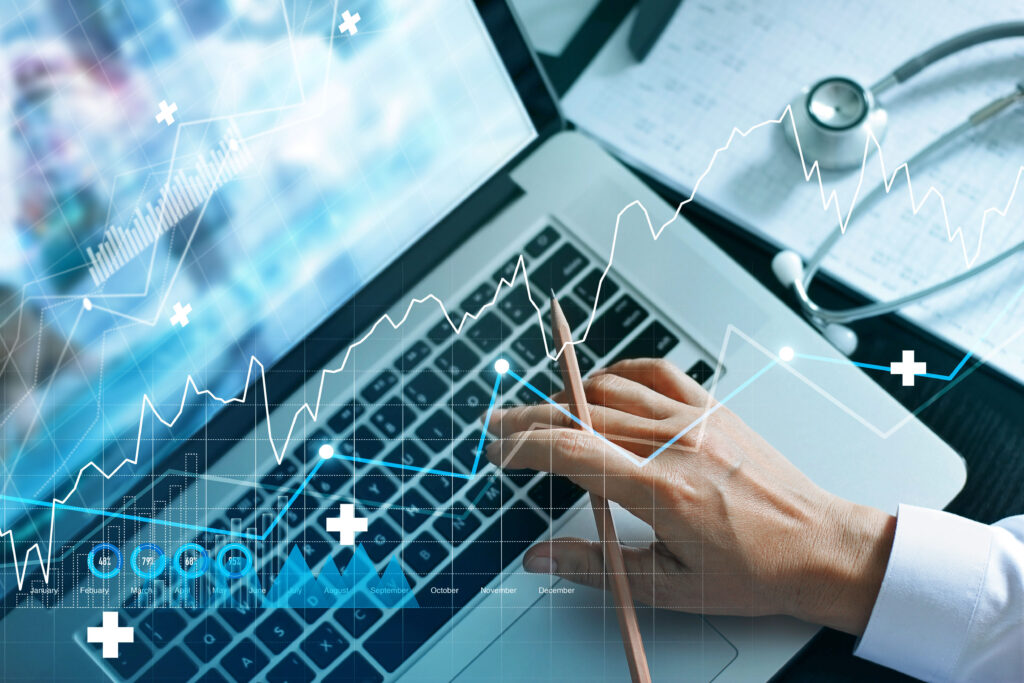
Even after many months of hype and hope, there’s wide divergence of opinions around how advanced technologies can best make a consequential difference in healthcare. While achieving some immediate gains in relieving staff burdens and automating some administrative tasks, the question remains – what’s the best hope for a big technology payoff?
Initial evidence suggests that some systemic reforms are necessary to enable these technologies to achieve maximum impact. We’re talking about streamlining operations, optimizing resource allocation and improving patient engagement to best leverage the latest technologies. And there’s potential benefits to unlock in increasing price transparency, implementing value-based care models, better measuring performance, enhancing care coordination and promoting preventive care strategies.
These are the areas in which artificial intelligence, machine learning and data science can return consequential benefits in healthcare. And we’re at a crucial pivot point for these technologies to deliver – health outcomes in the United States are worse than those of other developing countries, even though the nation is facing rising costs that pose multifaceted risks because of unaffordability and underutilization of necessary medical treatments or preventative care.
Because implementing these advanced technologies will be a costly layer on top of existing information systems employed in healthcare, it’s crucial for the industry to proactively plan how best to do so. Early examples of their use, however, show great promise for the future.
Analytics can lead the way
For example, the integration of big data analytics in healthcare demonstrates its potential to reduce costs while improving patient outcomes. Predictive analytics, machine learning and data mining techniques give clinicians opportunities to enhance decision-making.
By analyzing large datasets, healthcare providers can identify trends, optimize resource allocation and implement preventive measures, ultimately leading to more efficient care delivery and cost savings.
Taking further advantage of these capabilities requires collaboration among stakeholders, which include policymakers, healthcare providers, insurers and patients, with the downstream result of more efficient care delivery and cost savings.
Toward this end, the Centers for Medicare & Medicaid Services and policymakers can invest in research and grant-based initiatives to explore and test innovative strategies that, once validated, can be scaled for broader implementation, which holds the promise of substantial improvements in outcomes and effectiveness.
Rural health initiative
One such initiative was the Improving Health for At-Risk Rural Patients (IHARP), funded through innovation grants from CMS. The program aimed to enhance healthcare delivery and outcomes for at-risk rural populations by implementing new strategies and improving care coordination. by doing so, it succeeded in saving $1.2 million in its first 14 months of operation.
The foundation of IHARP’s success was its effective integration of machine learning models, statistical analysis and data mining techniques, thus enabling the extraction of valuable insights from complex data sets, which helped drive informed decision making, thus enhancing overall outcomes.
The program used descriptive statistics – such as means, frequencies, ranges and standard deviations – to determine all clinical outcome measures. Generalized estimating equation models were used to assess the differences in outcomes between the pre- and post-intervention periods for collaborative and typical care.
Savings from the program stemmed from decreased utilization of healthcare services, such as fewer doctor visits, reduced emergency department admissions and decreased hospitalizations. Also, there were notable improvements in health outcomes, such as lowered blood pressure levels and enhanced diabetes management among patients.
COVID-19 and other use cases
Advanced technologies have also had positive results in other anecdotal uses. For example, for the purposes of classifying chest X-ray images as either COVID-19 positive or normal (healthy), a model was developed by incorporating deep learning techniques such as deep feature extraction, fine-tuning of pre-trained convolutional neural networks (CNN) and end-to-end training of a newly developed CNN model. The deep learning model showed potential, achieving an accuracy score of 98 percent in detecting COVID-19 through chest X-rays.
Another study conducted in the Netherlands used machine learning models to identify an optimal distribution of COVID-19 vaccines, important during the early stages of vaccine distribution because of limited availability. It used an allocation principle that focused on targeting subgroups that would yield a significant reduction in bad outcomes.
Predictive analytics also can play a role in boosting systemic efficiency. For example, a study highlighted the effectiveness of deep learning models in predicting conditions like diabetic retinopathy from medical images. Additionally, the use of electronic health records systems holds great promise for advancing clinical research and enhancing clinical decision-making.
A machine learning model used an unsupervised deep-feature learning method to create a general-purpose patient representation from EHR data, supporting clinical predictive modeling. This approach delivered promising results for predictive performance for conditions such as severe diabetes, schizophrenia and various cancers. This bodes well for applying deep learning to EHR data to generate patient representations that improve clinical predictions, offering a robust machine learning framework to enhance clinical decision support systems.
One predictive risk model highlighted in a study by the National Institute on Minority Health focused on assessing stroke risk in adults with migraine accompanied by aura. The research involving 429 participants in their 50s, identified five key risk factors – diabetes, age over 65, heart rate variability, high blood pressure and gender. Stroke rates were significantly higher in the high-risk group (34 percent), compared with the low-risk group (3 percent).
Future potential for analytics
Predictive analytics also can make an impact on utilization and other non-clinical impacts on care. For example, studies have shown that predictive analytics can effectively identify patients at higher risk of readmission, enabling healthcare providers to implement targeted interventions and reduce admission rates.
The look-ahead capabilities of analytics also can better prepare clinicians and agencies tasked with preparing the country for medical eventualities. For example, a recent article emphasizes the application of artificial intelligence, machine learning models and healthcare informatics in proactively identifying potential pandemics long before they emerge. Researchers used clinical healthcare data and ICD-9 and ICD-10 codes to detect the spread of COVID-19. This innovative approach aims to enhance preparedness and response strategies, ultimately safeguarding public health using data science and machine leaning solutions.
A recent article discusses how predictive analytics models are being used to estimate life expectancy for patients with diabetes. Researchers analyzed various health data to identify key factors that influence longevity, including age, treatment adherence and comorbid conditions. By employing these models, healthcare providers can better tailor treatment plans and interventions to improve patient outcomes and extend life expectancy, thus enhancing chronic disease management.
In addition, predictive analytics can assist with early detection, which can improve planning and resource utilization. For example, one article examined an AI algorithm developed by researchers in South Korea to detect breast cancer using global data. AI achieved a higher accuracy rate (88.8 percent) than radiologists (75.3 percent). Radiologists supported by AI had an accuracy rate of 84.8 percent.
The synergy between AI and health data
Linking health informatics with machine learning algorithms and artificial intelligence can help process and transform healthcare data in an efficient manner, experts contend.
The efficacy of these strategies is backed by a growing body of research and development for real-world applications, with numerous studies and case examples demonstrating the positive impact of predictive analytics across various healthcare settings.
Some caveats still exist, however. It's important to recognize that the impact of these technologies can differ depending on specific use cases, data quality and implementation methods. Additionally, ethical and privacy issues, as well as the need for responsible data handling, remain critical concerns that demand careful consideration and further research.
To fully harness the potential of AI and ML, experts and stakeholders in healthcare, as well as government policymakers must collaborate in addressing challenges and solving urgent challenges. Using these technologies has the potential to reduce overall healthcare costs for providers to achieve better health outcomes by early detection and prevention.
Bhavini Kaneria is a senior analytics manager and leader in informatics, machine learning and artificial intelligence. She has worked extensively on various research-based healthcare projects in Medicare, Medicaid dual eligible special needs plans and community care. She also worked on the IHARP project as a research assistant performing prediction, forecasting and data analysis. For more information, contact bhavini.kaneria@gmail.com.