The role of data management and digitization in value-based care
As healthcare systems shift to value-based care models, the need for comprehensive and accurate patient data becomes paramount.
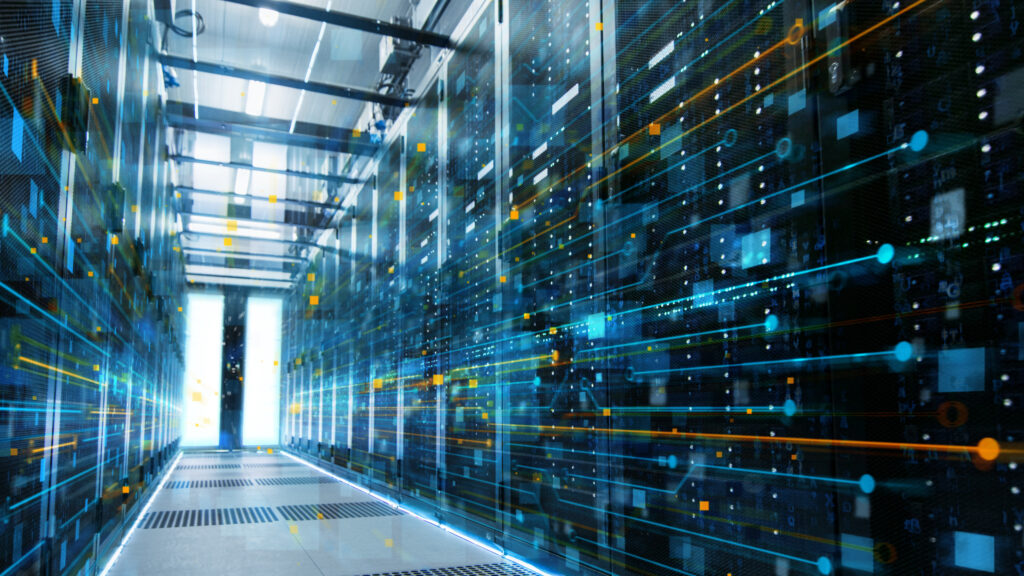
Value-based care adoption is accelerating, as providers, payers, employers and government entities become more comfortable with payment models beyond traditional fee for service.
The number of patients treated by physicians under value-based care (VBC) payment models “could roughly double in the next five years, growing approximately 15 percent per year,” McKinsey forecasts in a December 2022 report it entitled, “Investing in the new era of value-based care.”
More recently, Bain & Company wrote that value-based care “stakeholders are doubling down on their commitment as healthcare spending outpaces growth in the gross domestic product and [the Centers for Medicare & Medicaid Services] leans further into VBC models. This momentum is likely to accelerate as we see further regulation, more data from early adopters and more capability-enhancing technology.”
Indeed, it has become increasingly essential that payers and providers have comprehensive, accurate patient data – along with the ability to analyze and share that data – to support these new models of care. Integration of digitized data with structured and external datasets can provide a holistic view of the patient that enables actionable insights, enabling payers and providers to reduce costs and improve care quality under VBC contracts.
Effective support for VBC
However, the shift to value-based models alone will not transform healthcare. Achieving that level of change will require numerous data digitization and infrastructure capabilities. About 80 percent of primary care providers are interested in VBC reimbursement models, “but report technological and administrative constraints as the biggest barriers,” according to Bain. “This need has created high demand for both technology and services that enable VBC.”
For healthcare organization to fully realize the cost savings and quality improvement goals of VBC, they must be able to do the following:
- • Utilize a data-driven digital infrastructure that supports a multi-stakeholder "network of networks," which includes different payment capabilities.
- • Attain data interoperability to facilitate permissioned data-sharing.
- • Build longitudinal healthcare records that patients can supplement with their personal data.
- • Draw insights from patient data and external data sets to guide clinical decision-making.
- • Create user-friendly, data driven workflows for various healthcare stakeholders.
Healthcare data is still messy
Building the capabilities to take full advantage of the data needed to drive value-based arrangements remains a challenge for many healthcare organizations. Much of this difficulty is a result of the unique and messy nature of healthcare data.
Here are some of the problems:
Unstructured data. As much as 80 percent of healthcare data is unstructured, according to some industry estimates, which means it’s not in a form that easily lends itself to digital analysis. Images, audio, video, notes, charts, faxes and free-form text all are examples of unstructured data. While unstructured data contains valuable and relevant patient information, too often it provides minimal value to healthcare organizations because it rarely is digitized and combined with other forms of data sets.
Failure to meet data standards. There are several healthcare-industry data standards, including HL7, X12 EDI, FHIR, NCPDP, CCLF and CCDA – for processing patient data. Unfortunately, these standards are not consistently adhered to in different organizations. Even worse, data standards frequently are nonexistent for many patient data sets, such as data from devices, wearables and social determinants of health (SDoH).
Little or no external data utilization. When combined with transactional and clinical data sets, external data sets (such as those maintained by community-based organizations and government entities) can provide a 360-degree view of the patient that enables healthcare organizations to identify and proactively address SDoH-related issues and other factors that impact access to care.
Limited access to real-time data. In general, healthcare organizations are unable to access patients’ longitudinal health records across systems or payment information in real time. This can undermine point-of-care decision-making and lead to delays in the revenue cycle.
Inadequate infrastructure. As the Bain data suggests, most healthcare provider organizations lack an IT infrastructure that can support a “network of networks” model.
Data redundancy. Duplicate data errors are common in healthcare electronic health records, even within the same data set.
Using artificial intelligence and machine learning
Patient data sets typically are fragmented across different systems, requiring them to be digitized and combined with structured and external data sets to create the 360-degree view of a patient’s health, necessary for clinically sound decision-making in value-based arrangements. Artificial intelligence (AI) and machine learning (ML) can be deployed to digitize data and reduce errors. AI and ML help better automate tasks and decision-making processes while facilitating bidirectional integration between information systems.
AI-based technologies such as natural language processing (NLP) and computer vision can drive data digitization by converting unstructured information from notes and images into structured data that can inform care delivery and coordination. ML algorithms also can help reduce claims denials by improving error-detection in billing and coding. Further, AI and ML tools accelerate the processing of large data sets, helping to inform precise and comprehensive value-based risk forecasting and providing recommended actions to improve patient outcomes.
A cloud-based data infrastructure
Healthcare organizations building detailed longitudinal health records require a robust data infrastructure. It must be able to support unstructured data, which comprises the vast majority of EHR data. In addition, the data infrastructure must be able to do the following:
- • Process standard (HL7, FHIR and other) data sets, non-standard data sets and external data sets.
- • Implement a data digitization process that tags and merges this data with the rest of the data while using proper categorization.
- • Deploy an enterprise master patient index algorithm to tie disparate patient data records into a unified patient record.
- • Leverage APIs to expose patient records using DaaS (Data as a Service) and in the platform infrastructure through a Platform as a Service (PaaS)/Software as a Service (SaaS model, running on a modern application stack offering micro-services.
Provider and payer organizations that fulfill these requirements will be able to digitize data, synthesize different types of data sources, identify errors and seamlessly integrate new data sources.
The effectiveness of VBC heavily depends on the ability of healthcare organizations to successfully digitize data. By ensuring easy access to accurate, comprehensive and up-to-date longitudinal patient records, they can deliver better patient outcomes at lower costs.
Rahul Sharma is CEO of HSBlox, an Atlanta-based technology company.
To read more on value-based care, check out the July COVERstory.