Unleashing AI and ML: Transforming the healthcare landscape
How the industry can navigate challenges with advanced technology for improved patient outcomes and efficient healthcare management.
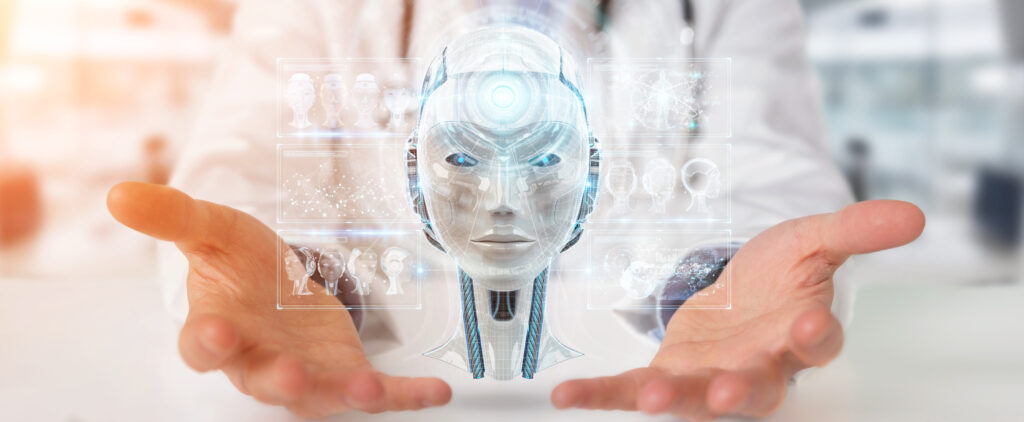
The healthcare industry faces myriad challenges that impact the delivery, accessibility and quality of healthcare globally. Rising costs and affordability are important factors, as are health disparities related to age, population group, socioeconomic status, race, availability of insurance coverage, healthcare facilities and medical personnel.
Fortunately, technological advancements, including artificial intelligence (AI) and machine learning (ML), address these critical issues. When properly utilized, these technologies help healthcare providers detect and diagnose a variety of conditions, including Alzheimer’s disease, heart disease, cancer, tuberculosis, and skin and liver diseases, with increased accuracy, leading to better patient outcomes.
In addition, advanced technologies provide data models to leverage patient information, including electronic health records, lab results and vital signs. In addition, they reduce the burden on healthcare staff, improve patient engagement and enhance access to better healthcare outcomes. From a business perspective, AI and ML technology aids in resource allocation, cost optimization, processing of claims, automating eligibility, fraud detection and prevention, and numerous other functions.
To take advantage of these opportunities, it’s important for healthcare professionals to work closely with data scientists and other technology experts to capitalize on the benefits of these technologies.
Understanding the challenges
To be able to address today’s healthcare challenges, it’s necessary to understand why they are happening. Many factors contribute to healthcare’s rising costs, including inflation, a pay-per-service model, the lack of standardized costs, procedure costs, expensive new technologies, and population trends such as the increase in chronic diseases and an aging population.
In addition, many people often have complex needs that require coordination with multiple healthcare providers including Medicare and Medicaid. Without coordinated care and integration, patients find themselves with gaps in service, fragmented service and unique challenges when managing chronic conditions. These become more apparent within the aging population, where today there is an increased prevalence of multiple conditions, including diabetes, cardiovascular conditions and cancer.
Access to affordable, quality healthcare continues to be a serious problem, particularly for underserved populations, low-income individuals and those in rural areas. While access may be most simply understood as an individual’s proximity to and abundance of healthcare facilities, it includes other factors such as insurance coverage, wait times for care, and the abundance of appropriate healthcare providers.
Even with access to healthcare, there are clear disparities in health outcomes when considering factors like race and ethnicity, socioeconomic status and even geographic location. These are further exacerbated among impoverished individuals and communities that have inadequate housing, suffer from food insecurity or have limited access to transportation.
Beyond these generic issues, there are severe global healthcare challenges, made all too clear by the COVID-19 pandemic. In addition to fears of another global flu pandemic, additional threats include growing antibiotic resistance and outbreaks of other infectious diseases such as Ebola. How well global healthcare systems will respond to threats like these in the future will depend on their preparedness and willingness to collaborate.
Technological challenges
Advances have been made in data sharing and interoperability in the healthcare industry, enabling healthcare providers to facilitate care coordination and ensure a comprehensive understanding of beneficiaries’ healthcare needs. Still, several issues need to be addressed. Among them are the privacy concerns that can complicate seamless information exchanges and hinder effective care coordination and quality of care for patients.
There are also complex obstacles connected to health information overload. While access to health information online is a positive gain for the industry, the challenge is mining and accurately interpreting the vast amount of raw data to make it available and understandable to healthcare providers and patients.
Many of the daunting problems facing the industry can be addressed through technologies such as AI and ML. When dealing with patients with severe or chronic conditions, AI algorithms have significant potential in the analysis of medical images to detect abnormalities and assist in diagnosing diseases that may otherwise be difficult to diagnose before they progress further.
For instance, breast cancer is a leading cause of death for women, but between 10 percent and 30 percent of cancers may be missed in mammograms. In a 2020 study published in The Lancet, researchers from South Korea developed an AI algorithm to detect breast cancer using data from around the world. When the AI’s performance was compared with that of radiologists, the AI had an 88.8 percent accuracy rate compared with the radiologists’ rate of 75.3 percent. When aided by AI, however, the radiologists’ accuracy rate jumped to 84.8 percent. In addition, by analyzing data about medical history, demographics and advanced image assessment, machine learning supports the early detection of conditions such as cancer, strokes and cardiovascular disease.
Researchers in the medical field are also examining how AI models could be used to leverage patient data, including those included in electronic health records, lab results and vital signs to predict disease progression and patient outcomes and identify high-risk individuals who may require proactive intervention.
An article in the Journal of Medical Internet Research examines some of the objectives for AI in this area – they include risk scoring (for example, predicting disease development risk), subtyping (grouping patients based on relevant factors) and pathway discovery (identifying frequent patterns in patient trajectory). This application of AI and ML could also support targeted public health interventions directed at high-risk populations or in specific geographical areas identified using AI clustering algorithms.
Several additional studies have looked at the predictive abilities of ML for other specific diseases. One study’s method predicted COVID-19 progression with an accuracy of 90 percent to 98 percent, and other studies demonstrated that ML can be used to predict the progression of both multiple sclerosis and Alzheimer’s disease.
AI is also accelerating new drug discovery processes through target identification, high-fidelity molecular simulations and drug property predictions, reports Harvard’s Bill of Health, all of which can help reduce both the time and costs associated with developing new drugs.
In addition to these potentials, a 2021 review of AI in the pharmaceutical industry includes studies using AI to improve clinical trial design, quality control and the business management of pharmaceuticals.
Remote monitoring and telemedicine have already proved invaluable during the pandemic and have the potential to address service gaps for those in remote and underserved communities. Taking this technology one step further, AI-powered remote monitoring devices and wearables could collect real-time patient data of patients who are continuously monitored, thereby supporting the detection of health issues. Telemedicine platforms integrated with AI can facilitate remote consultations, allowing healthcare providers to diagnose and treat patients from a distance.
Fraud, security and privacy
There’s no doubt that data security and privacy are ongoing issues today, particularly in the healthcare industry, where it’s essential that the Health Insurance Portability and Accountability Act (HIPAA) laws are protected. AI can enhance data security by detecting and preventing potential cybersecurity threats and unauthorized access to sensitive healthcare information, and AI algorithms can help identify and protect against data breaches, ensuring compliance with privacy regulations and safeguarding patient confidentiality.
By analyzing large volumes of data to identify patterns and anomalies, AI algorithms can flag potentially fraudulent activities. Several countries – including Greece, India, and South Korea – are already using AI and ML to prevent and detect healthcare fraud. Models can be developed to detect unusual billing patterns, identify potential fraudulent providers and automate the identification of suspicious claims for further investigation.
A working collaboration
The key to integrating the vast potential of AI and ML in ensuring better health outcomes lies in bringing together those working in these two distinct industries.
Data scientists are technical experts who understand the necessity of AI and ML. They need healthcare professionals to explain the domain knowledge to them to ensure scientists can create and build the models needed to solve the problems.
For that to happen, it’s essential to train more people with technology and healthcare domain knowledge. After they have that knowledge and the understanding of the necessary tools, AI and ML can be harnessed in the healthcare industry to do everything from analyzing patient data to predicting disease progression and patient outcomes. General knowledge data scientists might be able to survive in other industries, but in the healthcare industry, they need additional education, training and experience.
To leverage the full potential of AI and ML, it is essential for different experts and stakeholders within healthcare and government policymakers to collaborate to find ways to address challenges and solve pressing problems. Innovative healthcare projects already show it is possible to utilize data and healthcare expertise to address complex challenges with cooperative effort, even without the power of AI and ML.
One such project was launched in 2014 by the Centers for Medicare & Medicaid Services, called IHARP (Improving Health for At-Risk Rural Patients—Carilion New River Valley Medical Center). The project received a CMS Health Care Innovation Award to improve medication therapy management for Medicare and Medicaid beneficiaries and patients in 23 underserved rural counties in southwest Virginia.
The project used a collaborative care model, embedding pharmacists as part of patients’ primary care teams to work jointly to develop individual patient care plans. The pharmacists were trained in chronic disease management protocols, with the aim of improving clinical outcomes and satisfaction with care and decreasing healthcare costs for high-cost, high-risk patients with multiple chronic diseases. By incorporating medication management and home medical visits, researchers were able to reduce hospital and ED visits, which led to more positive outcomes.
According to the CMS report, “Through care coordination and shared access to electronic medical records, the project enabled pharmacists to participate in improving medication adherence and management, resulting in better health, reduced hospitalizations and emergency room visits and fewer adverse drug events for patients with multiple chronic diseases.”
The healthcare industry is already working to address complex challenges through data science and collaboration. AI and ML are simply tools that can be applied to the current challenges and problems within the system to provide improved healthcare and patient outcomes.
As long as policymakers, healthcare providers, researchers and stakeholders in the healthcare industry are willing to work together and understand that challenges can vary across different regions and healthcare systems, it is possible to launch innovative, life-saving projects with the use of AI and ML technologies. With ongoing monitoring, adaptation and continuous improvement, it will be possible to create a far more sustainable and effective healthcare system.
Bhavini Kaneria is a senior analytics manager and leader in informatics, machine learning and artificial intelligence. She worked on the IHARP project as a research assistant, performing data analysis, reporting, prediction and forecasting work. She can be reached at bhavini.kaneria@gmail.com.