Unlocking the value of distributed health data for machine learning
The use of federated architecture enables distributed approaches that offer safer approaches to support analytics and healthcare research.
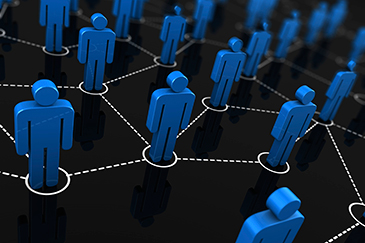
With the digitization of health data and the application of machine learning and analytics, researchers, clinicians and administrators are developing and adopting new tools to improve patient outcomes, reduce healthcare delivery costs and accelerate drug development pipelines.
However, challenges accessing health data limit practitioners’ ability to unlock the opportunities of AI in healthcare.
Impractical efforts in data centralization
Health data is generated across thousands of institutions and clinics across borders (and even within institutions), and is produced by a wide range of devices, staff and departments. This creates problems in trying to apply machine learning to it.
Outside of healthcare, the primary approach to applying machine learning and analytics on distributed data is to first centralize the data in a data lake or data warehouse. However, three unique characteristics of health data have rendered centralization to be frequently impractical or even impossible: sensitivity, volume and interoperability.
Sensitivity. Most countries have regulations limiting the usage of personal data, and many have supplemented their regulations with further guidance on protecting personal health information. GDPR in the EU and HIPAA in the U.S. severely limit the sharing of health data between institutions or across borders without express consent. Health data custodians also have their own privacy and security protocols, as well as concerns with sharing intellectual property which give them a competitive advantage.
Health institutions and product developers have traditionally managed these trust barriers through a combination of technical de-identification and legal means, but each has significant limitations. Because of the complexity and cost associated with sharing health data,many potentially high-value initiatives are slow or impossible to get off the ground — a major loss for researchers and patients.
Volume. The explosion of health data unlocks new opportunities for researchers to improve existing models with new features, and build new predictive models for diagnostics, precision medicine and real-world evidence. But the promise of boundless health innovation driven by the sheer volume of digital health data must be tempered by the practical implications of moving and storing copies of these massive data sets.
The compute time and costs required to centralize data for machine learning and analytics severely restrict health AI innovation.
Interoperability. A historical lack of data standards in healthcare also creates challenges for data aggregation across sites. Hospital electronic health record (EHR) systems are designed to optimize hospital operations and comply with local rules and regulations, not to facilitate data sharing. Converting existing data to a standard format so it can be aggregated across systems is both time-consuming and costly.
Efforts such as the Fast Healthcare Interoperability Resources (FHIR) open-source framework in the U.S. are underway to establish and enforce better health data standards. Challenges with adoption still exist outside the U.S., and interoperability does not solve the challenges of sharing sensitive data at scale.
Effects of distributed health data
History has proven that barriers to sharing health data for machine learning and analytics hinder the overall progression of AI in healthcare.
The cost and complexity of consolidating data make centralization impractical, while distributed systems place significant limitations on the ability to extract insights from remotely stored data. Simply put, the existing approaches to using machine learning and analytics on health data are no longer working and it is time for a new approach.
With the continued explosion of digital health data, health AI requires data scientists to explore new approaches beyond centralizing the data. In the federated future, health data will not be moved, and teams will be able to unlock insights from health data around the world while preserving patient privacy.
Unlike traditional machine learning, federated learning and analytics enable data scientists and researchers to train models and do analytics without bringing the data together.
How it works
A central federated learning server hosted by a trusted party transmits training instructions to each hospital’s data server, where a local model is trained. Local model parameters are sent back to the federated learning server, where they are aggregated into one global model. The nature of federated learning makes it the ideal solution for health AI, because data never moves and it is privacy-preserving.
Thinking back to the three unique characteristics of health data that make data centralization impractical, federated learning can help solve these challenges by making an impact through:
Sensitivity. Institutions continue to require patient consent or a legal basis to share data for a specific purpose. However, because data does not move and federated learning is privacy-preserving, data sharing becomes much easier, enabling compliance with regulations like GDPR and HIPAA.
Volume. Because data does not move in a federated architecture, the costs and compute time for moving and storing large volumes of data are moot. Aggregate data like model parameters still move between servers, but this volume is minuscule, compared with the raw data sets.
Interoperability. While federated learning does not solve challenges with data standardization and interoperability, in a federated architecture all data must be standardized for model training to execute properly. Existing efforts to drive adoption of FHIR standards will continue to benefit everybody, even as teams transition to a federated architecture.
Although the health AI ecosystem is still in early experimentation with federated learning and analytics, there is growing interest in the opportunities that it may unlock. Already, the technology has been applied across a number of use cases, such as predictive diagnostics, precision medicine and drug discovery, to name a few.
In this future of improved access, researchers and data scientists will be able to leverage data from connected medical devices without moving data to centralized servers. Health application developers will recognize new revenue opportunities by enabling machine learning and analytics across their data networks, while their partners retain full control over their own data. And all stakeholders across the health ecosystem will reap the benefits of new and better insights from AI to efficiently deliver better patient outcomes.
While barriers to the adoption of privacy-preserving tools for increased data access abound, crucial elements of healthcare delivery suffer, including diagnostic accuracy, patient outcomes, pipeline development speed, drug approval time and more, all at the costs of patients and an overburdened and understaffed healthcare ecosystem.
Dr. Bryce Pickard is partnerships director for integrate.ai
Also see: Federated learning feeds more data to support AI processes