Why AI must increasingly power cybersecurity in healthcare
Because organizations are seeking increased connectivity, security professionals need AI to provide more capabilities to respond faster and effectively.
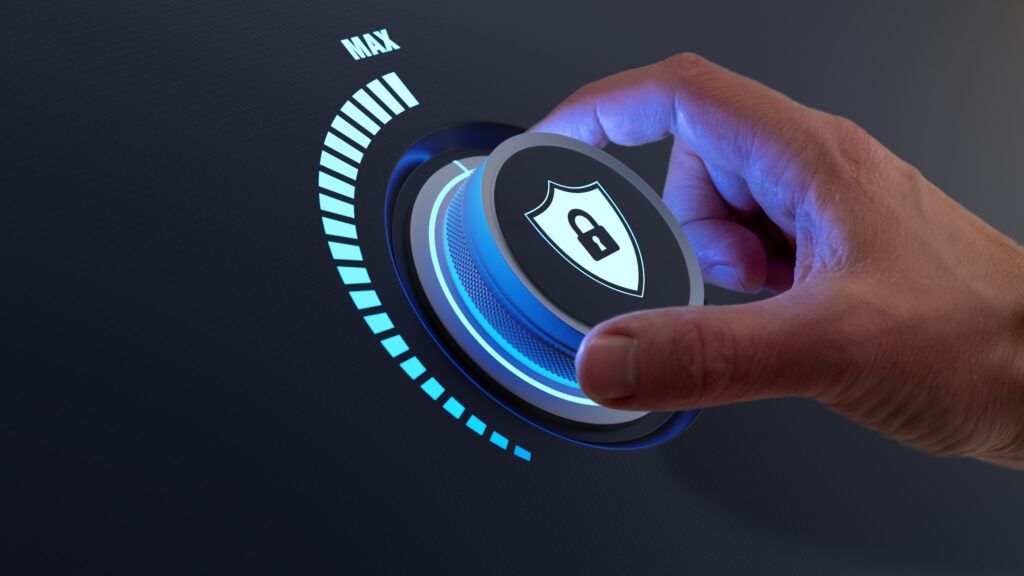
Cybersecurity is crucial in healthcare because organizations handle highly sensitive patient information and support systems essential to public health. While the industry has enhanced care delivery with increased digitization through tools like electronic health records, telemedicine and IoT medical devices, this has also increased its vulnerability to cyberattacks.
The healthcare industry is well-acquainted with data breaches and cyber threats, which pose risks to both patient privacy and the integrity of healthcare operations. As cyberattacks continue to evolve, methods that are effective for protecting organizations and their systems today can quickly become obsolete. There has been a 50 percent year-over-year increase in the number of cyberattacks.
Stolen healthcare records can fetch nearly 10 times as much as stolen credit card numbers on the dark web. Additionally, the cost of addressing a healthcare data breach is nearly three times resolution costs in other industries, averaging $408 per compromised healthcare record, compared with $148 for the average non-healthcare record. These figures highlight the significant financial and security challenges healthcare organizations face in managing data breaches.
The perils of connectivity
As healthcare organizations leverage technology to improve efficiency, they also expose themselves to increased cyberattack risks. Hackers often target third-party vendors with the goal of gaining access to larger connected organizations. Such access through a third-party involved Target Stores, which was compromised via an HVAC company's login credentials, enabling hackers to access customer data and eventually expand their reach into other systems.
This Target Stores attack illustrates the risks of interconnected systems and the importance of securing third-party relationships. In this breach, hackers initially tested their malware on a few cash registers before uploading it to most of the company's point-of-sale systems, starting on Nov. 27, 2013. They then stole data from around 40 million credit and debit cards. By the time Target responded to alerts from its FireEye software, hackers had accessed sensitive financial data, leading to the compromise of thousands of individuals' financial records.
Healthcare organizations have many priorities, and they often lack the time, budget and resources to effectively defend against cyberattacks. When they do invest in cybersecurity, it is typically aimed at preventing attacks from less sophisticated hackers. In the past, many attacks were attempted by unskilled individuals who simply wanted to explore an organization's systems. Now, however, there’s an increasing number of more financially motivated cyberattacks, such as ransomware.
The increasing interconnectivity of systems and the rise of technologies like IoT devices with limited security defenses expand the attack surface. This leaves organizations vulnerable to financial losses, public safety risks and potential harm to supply chains, ultimately putting lives at risk.
The capabilities of AI in cybersecurity
Artificial intelligence has emerged as a transformative technology in recent years, improving existing methods and making them more capable of thought-like processes. Artificial intelligence and machine learning are revolutionizing cybersecurity with their ability to enhance defenses against increasingly sophisticated threats.
AI-powered cybersecurity can detect, analyze and respond to threats in real time by analyzing vast data sets to identify patterns and vulnerabilities. It monitors behavior patterns, establishes baselines and flags unusual activity to prevent unauthorized access. AI also can prioritize risks and detect malware or intrusions early. By automating repetitive tasks, AI frees up resources and reduces human error in security processes.
AI in cybersecurity will not replace security professionals, but it will support them by analyzing large volumes of data, recognizing patterns and generating insights that would take hours or weeks with traditional analytical approaches.
Previously, security relied on signature-based detection tools, which were effective against known threats but inadequate for zero-day or unknown attacks, often leading to false positives. Traditional methods also relied on manual analysis, which was time-consuming and prone to error. AI can address these limitations, enhancing cybersecurity efficiency and effectiveness as it continues to evolve.
AI can be extremely useful in threat detection and prevention. AI, especially machine learning and deep learning, analyzes vast amounts of data to spot patterns and anomalies that signal potential cyber threats. It can detect malware, recognize unusual behavior and predict future attacks based on past attacks, enabling proactive defense.
For example, AI can enhance automated threat response and incident management by accelerating reactions to security incidents. In the event of a breach or attack, AI can automate response protocols by quickly isolating affected systems, blocking suspicious IP addresses and restricting access, all the while sending real-time alerts to security teams.
Smart healthcare technology has become a key area of research because of the rapid growth of biomedical data and the healthcare community. The integration of cloud computing and Internet of Things (IoT) paradigms has driven the development of smart healthcare systems capable of diagnosing, monitoring and aggregating data. These devices are also exposed to cyber threats.
In furtherance of improving defenses, this paper introduces an AI-driven IoT eHealth architecture powered by deep neural networks, specifically an ICNN, to enhance accuracy and efficiency in healthcare data analysis. It explores the use of real-time data through the Grey Filter Bayesian Convolutional Neural Network (GFB-CNN), addressing challenges in service quality. The approach, tested on an extensive Mobile Health (MHealth) dataset, efficiently differentiates between healthy and abnormal heart signals, offering a cost-effective, fast solution for cardiac health monitoring, and it can be applied to cybersecurity in health data analysis for improved protection.
AI can enhance data encryption and privacy protection in healthcare by safeguarding sensitive information like personal health information and personally identifiable information. It can improve encryption through intelligent methods that adjust the extent of encryption based on data type, access and context, ensuring stronger protection. AI can support real-time data anonymization for research or analysis, preventing unauthorized access while still enabling data use in clinical studies or AI model training.
AI algorithms can detect unusual spikes in network traffic or unexpected patterns of user behavior that deviate from established norms, providing early warnings of intrusion attempts. Moreover, AI can be used for vulnerability management, behavioral biometrics for authentication, securing IoT devices, phishing and social engineering-based attack prevention.
Importance of AI in cybersecurity
Cybercriminal organizations are increasingly using machine learning, automation and AI to carry out large-scale, targeted attacks, resulting in the rise of the use of ransomware. AI and machine learning help security analysts process vast amounts of data, provide rapid insights, and filter out daily alerts and false positives, greatly enhancing team efficiency and productivity.
As sophisticated attack methods like polymorphic malware and "living-off-the-land" attacks bypass traditional defenses, newer approaches such as behavior analysis are gaining popularity. AI, when properly trained, can detect and respond to malicious behaviors much faster than humans, offering a powerful defense against evolving threats.
This paper explores the Zero Trust approach, emphasizing seven critical areas that healthcare systems must address to protect both individuals and organizations. The approach focuses on ensuring stronger security by verifying every user and device, minimizing risks and enhancing data protection. It highlights the importance of continuous monitoring, strict access control and adopting a robust cybersecurity framework to safeguard sensitive healthcare information.
Zero Trust is the term for an “evolving set of cybersecurity paradigms that move defenses from static, network-based perimeters to focus on users, assets and resources.” Zero Trust fundamentally challenges traditional security models by not granting implicit trust based on physical or network location, or asset ownership. This shift marks a significant departure from legacy authentication and security practices.
Challenges and considerations
While AI offers significant potential for enhancing healthcare cybersecurity, there are challenges to consider. One concern is data privacy, because AI systems require large amounts of data for training, raising issues around patient confidentiality.
There are also ethical concerns, particularly regarding decision-making processes, accountability and the transparency of AI systems. Additionally, healthcare organizations may face a skill gap, struggling to find and retain AI cybersecurity experts – the lack of qualified professionals could limit organizations’ ability to fully leverage AI for security purposes.
The use of AI has raised concerns about the quality of data required to train machine learning algorithms. Misinformation included in the training data can create more issues than it resolves. This focus on data has driven the need to expand security efforts beyond merely monitoring data files.
The American Hospital Association advises healthcare leaders to view cybersecurity as a critical issue that impacts more than just providers’ IT departments. It should be considered a key aspect of patient safety, enterprise risk and strategic planning. Cybersecurity should be integrated into the hospital's overall risk management, governance and business continuity frameworks to ensure comprehensive protection.
According to Info-Tech, today, “Most healthcare security architectures are perimeter-based and complex to manage.” The primary barrier to cybersecurity breaches is often the point of entry. Frequently, attacks are already embedded within a system, lying dormant until triggered. These attacks wait for specific conditions or actions to activate, making them particularly challenging to detect and prevent.
Organizations must track who accesses data and how, while also implementing effective backup and recovery processes. These practices ensure data security and availability, helping prevent unauthorized access and minimizing the impact of potential data breaches or losses.
Too often, a breach is not even recognized for months or years after it has happened. While it’s crucial for healthcare organizations to educate users about falling prey to malware, hackers are using increasingly sophisticated tools and methods. Even with security measures like multi-factor authentication, users can still be compromised if they fall for phishing emails or visit harmful websites. Adopting a proactive approach, such as integrating Zero Trust principles into the healthcare industry, is a crucial first step in minimizing the risk of unauthorized access.
There is high demand for individuals skilled in both cybersecurity and AI, as enterprises seek experts who can apply AI techniques to cybersecurity workflows. Roles such as data scientists, analysts and engineers with a cybersecurity background are crucial.
These positions require expertise in machine learning, deep neural networks, language modeling and behavior analysis, along with a solid understanding of cybersecurity principles. AI cybersecurity professionals must also be knowledgeable in network security, computer forensics, cryptography, malware detection and data protection.
Bhavini Kaneria is a senior analytics manager and leader in informatics, machine learning and artificial intelligence. She has worked extensively on various research-based healthcare projects in Medicare, Medicaid dual eligible special needs plans and community care. She also worked on the IHARP project as a research assistant performing prediction, forecasting and data analysis. For more information, contact bhavini.kaneria@gmail.com.